arXiv:2406.04512v1 Announce Type: new
Abstract: Arabic is known to present unique challenges for Automatic Speech Recognition (ASR). On one hand, its rich linguistic diversity and wide range of dialects complicate the development of robust, inclusive models. On the other, current multilingual ASR models are compute-intensive and lack proper comprehensive evaluations. In light of these challenges, we distill knowledge from large teacher models into smaller student variants that are more efficient. We also introduce a novel human-annotated dataset covering five under-represented Arabic dialects for evaluation. We further evaluate both our models and existing SoTA multilingual models on both standard available benchmarks and our new dialectal data. Our best-distilled model’s overall performance ($45.0$% WER) surpasses that of a SoTA model twice its size (SeamlessM4T-large-v2, WER=$47.0$%) and its teacher model (Whisper-large-v2, WER=$55.1$%), and its average performance on our new dialectal data ($56.9$% WER) outperforms all other models. To gain more insight into the poor performance of these models on dialectal data, we conduct an error analysis and report the main types of errors the different models tend to make. The GitHub repository for the project is available at url{https://github.com/UBC-NLP/distill-whisper-ar}.
Source link
lol
To Distill or Not to Distill? On the Robustness of Robust Knowledge Distillation
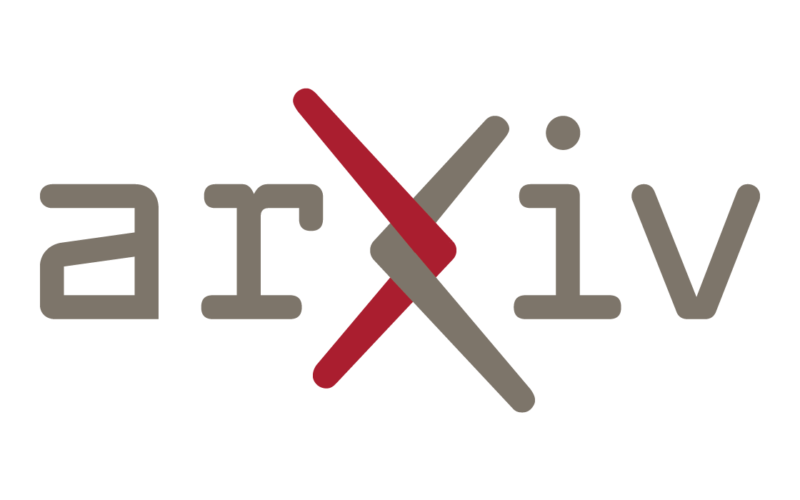