arXiv:2406.04532v1 Announce Type: new
Abstract: In the field of self-supervised depth estimation, Convolutional Neural Networks (CNNs) and Transformers have traditionally been dominant. However, both architectures struggle with efficiently handling long-range dependencies due to their local focus or computational demands. To overcome this limitation, we present MambaDepth, a versatile network tailored for self-supervised depth estimation. Drawing inspiration from the strengths of the Mamba architecture, renowned for its adept handling of lengthy sequences and its ability to capture global context efficiently through a State Space Model (SSM), we introduce MambaDepth. This innovative architecture combines the U-Net’s effectiveness in self-supervised depth estimation with the advanced capabilities of Mamba. MambaDepth is structured around a purely Mamba-based encoder-decoder framework, incorporating skip connections to maintain spatial information at various levels of the network. This configuration promotes an extensive feature learning process, enabling the capture of fine details and broader contexts within depth maps. Furthermore, we have developed a novel integration technique within the Mamba blocks to facilitate uninterrupted connectivity and information flow between the encoder and decoder components, thereby improving depth accuracy. Comprehensive testing across the established KITTI dataset demonstrates MambaDepth’s superiority over leading CNN and Transformer-based models in self-supervised depth estimation task, allowing it to achieve state-of-the-art performance. Moreover, MambaDepth proves its superior generalization capacities on other datasets such as Make3D and Cityscapes. MambaDepth’s performance heralds a new era in effective long-range dependency modeling for self-supervised depth estimation.
Source link
lol
MambaDepth: Enhancing Long-range Dependency for Self-Supervised Fine-Structured Monocular Depth Estimation
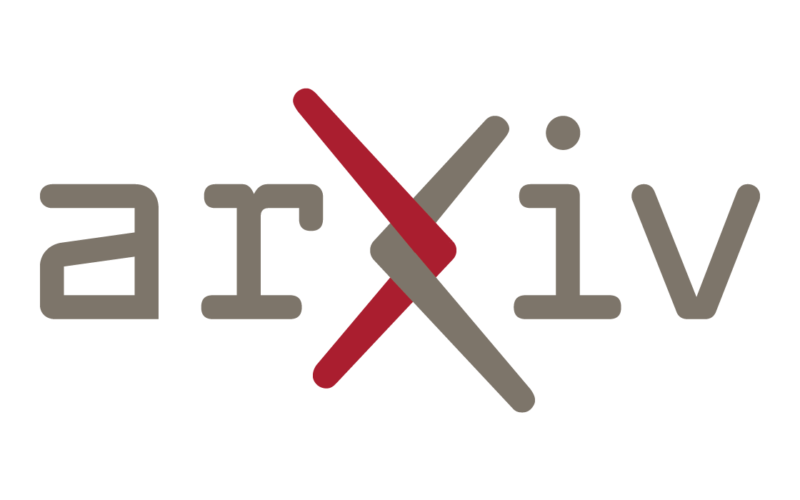