arXiv:2411.02448v1 Announce Type: new
Abstract: LLMs have demonstrated impressive proficiency in generating coherent and high-quality text, making them valuable across a range of text-generation tasks. However, rigorous evaluation of this generated content is crucial, as ensuring its quality remains a significant challenge due to persistent issues such as factual inaccuracies and hallucinations. This paper introduces two fine-tuned general-purpose LLM autoevaluators, REC-12B and REC-70B, specifically designed to evaluate generated text across several dimensions: faithfulness, instruction following, coherence, and completeness. These models not only provide ratings for these metrics but also offer detailed explanations and verifiable citations, thereby enhancing trust in the content. Moreover, the models support various citation modes, accommodating different requirements for latency and granularity. Extensive evaluations on diverse benchmarks demonstrate that our general-purpose LLM auto-evaluator, REC-70B, outperforms state-of-the-art LLMs, excelling in content evaluation by delivering better quality explanations and citations with minimal bias. It achieves Rank #1 as a generative model on the RewardBench leaderboardfootnote{url{https://huggingface.co/spaces/allenai/reward-bench}} under the model name texttt{TextEval-Llama3.1-70B}. Our REC dataset and models are released at url{https://github.com/adelaidehsu/REC}.
Source link
lol
Rate, Explain and Cite (REC): Enhanced Explanation and Attribution in Automatic Evaluation by Large Language Models
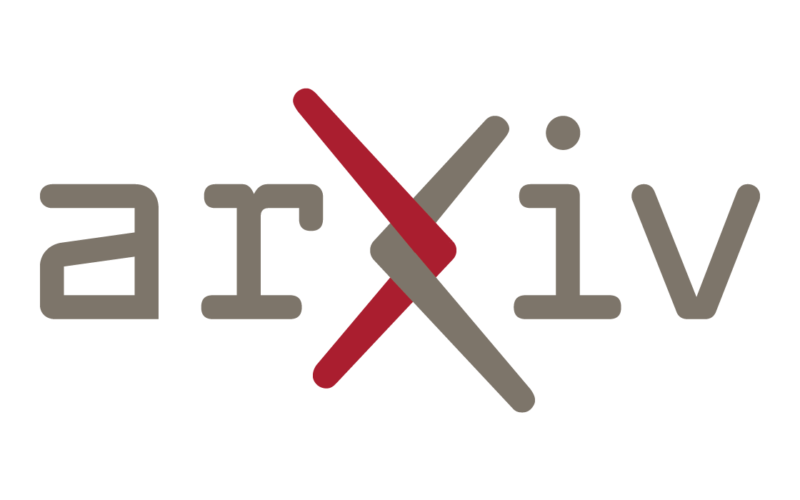