arXiv:2406.04425v1 Announce Type: new
Abstract: A fundamental problem in machine learning is understanding the effect of early stopping on the parameters obtained and the generalization capabilities of the model. Even for linear models, the effect is not fully understood for arbitrary learning rates and data. In this paper, we analyze the dynamics of discrete full batch gradient descent for linear regression. With minimal assumptions, we characterize the trajectory of the parameters and the expected excess risk. Using this characterization, we show that when training with a learning rate schedule $eta_k$, and a finite time horizon $T$, the early stopped solution $beta_T$ is equivalent to the minimum norm solution for a generalized ridge regularized problem. We also prove that early stopping is beneficial for generic data with arbitrary spectrum and for a wide variety of learning rate schedules. We provide an estimate for the optimal stopping time and empirically demonstrate the accuracy of our estimate.
Source link
lol
On Regularization via Early Stopping for Least Squares Regression
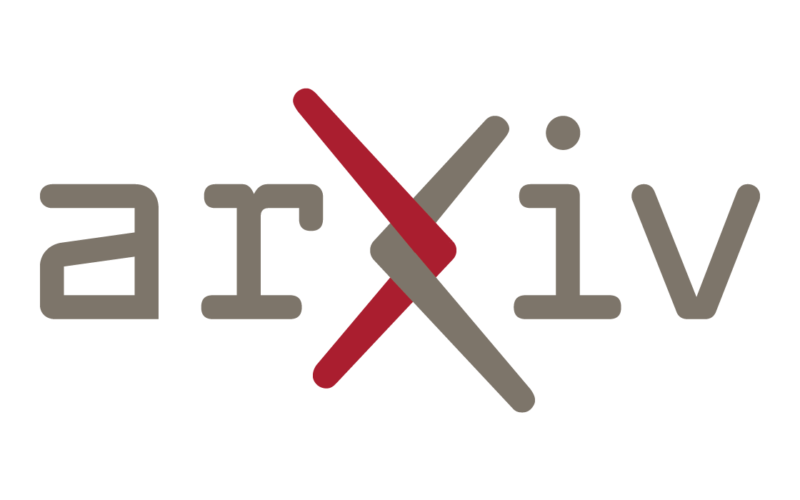