arXiv:2410.18161v1 Announce Type: cross
Abstract: Differentiating between Intestinal Tuberculosis (ITB) and Crohn’s Disease (CD) poses a significant clinical challenge due to their similar symptoms, clinical presentations, and imaging features. This study leverages Computed Tomography Enterography (CTE) scans, deep learning, and traditional computer vision to address this diagnostic dilemma. A consensus among radiologists from renowned institutions has recognized the visceral-to-subcutaneous fat (VF/SF) ratio as a surrogate biomarker for differentiating between ITB and CD. Previously done manually, we propose a novel 2D image computer vision algorithm for auto-segmenting subcutaneous fat to automate this ratio calculation, enhancing diagnostic efficiency and objectivity. As a benchmark, we compare the results to those obtained using the TotalSegmentator tool, a popular deep learning-based software for automatic segmentation of anatomical structures, and manual calculations by radiologists. We also demonstrated the performance on 3D CT volumes using a slicing method and provided a benchmark comparison of the algorithm with the TotalSegmentator tool. Additionally, we propose a scoring approach to integrate scores from radiological features, such as the fat ratio and pulmonary TB probability, into a single score for diagnosis. We trained a ResNet10 model on a dataset of CTE scans with samples from ITB, CD, and normal patients, achieving an accuracy of 75%. To enhance interpretability and gain clinical trust, we integrated the explainable AI technique Grad-CAM with ResNet10 to explain the model’s predictions. Due to the small dataset size (100 total cases), the feature-based scoring system is considered more reliable and trusted by radiologists compared to the deep learning model for disease diagnosis.
Source link
lol
Bridging the Diagnostic Divide: Classical Computer Vision and Advanced AI methods for distinguishing ITB and CD through CTE Scans
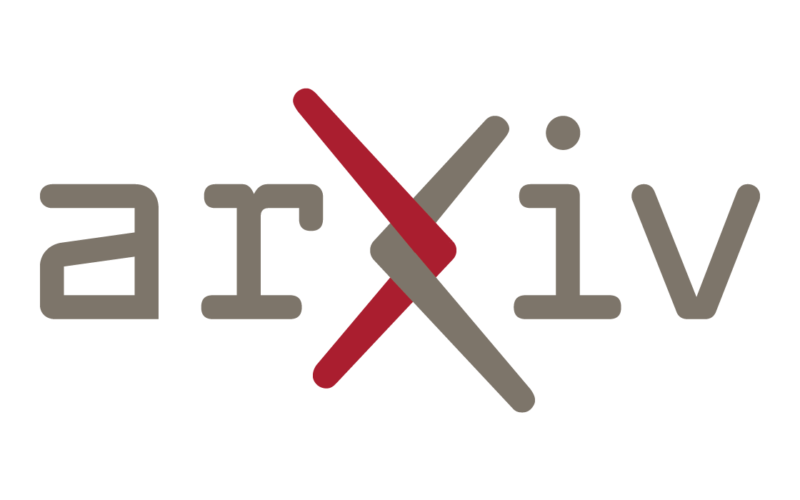