arXiv:2410.11080v1 Announce Type: new
Abstract: 3D Gaussian splatting has surpassed neural radiance field methods in novel view synthesis by achieving lower computational costs and real-time high-quality rendering. Although it produces a high-quality rendering with a lot of input views, its performance drops significantly when only a few views are available. In this work, we address this by proposing a depth-aware Gaussian splatting method for few-shot novel view synthesis. We use monocular depth prediction as a prior, along with a scale-invariant depth loss, to constrain the 3D shape under just a few input views. We also model color using lower-order spherical harmonics to avoid overfitting. Further, we observe that removing splats with lower opacity periodically, as performed in the original work, leads to a very sparse point cloud and, hence, a lower-quality rendering. To mitigate this, we retain all the splats, leading to a better reconstruction in a few view settings. Experimental results show that our method outperforms the traditional 3D Gaussian splatting methods by achieving improvements of 10.5% in peak signal-to-noise ratio, 6% in structural similarity index, and 14.1% in perceptual similarity, thereby validating the effectiveness of our approach. The code will be made available at: https://github.com/raja-kumar/depth-aware-3DGS
Source link
lol
Few-shot Novel View Synthesis using Depth Aware 3D Gaussian Splatting
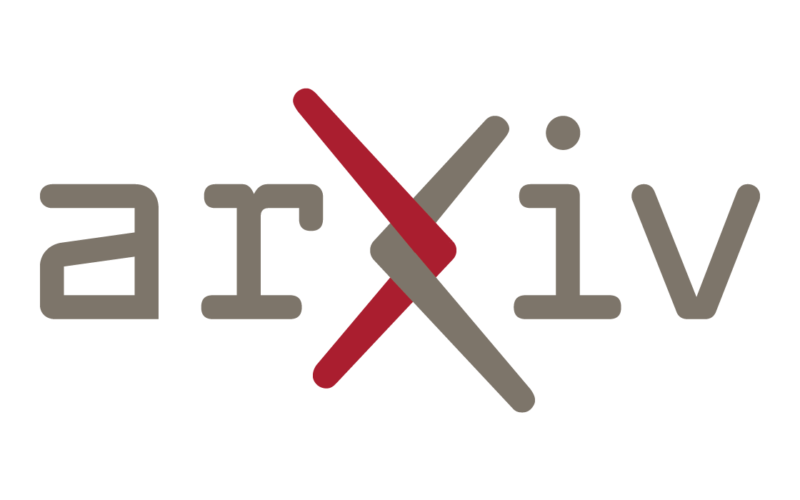