arXiv:2410.08375v1 Announce Type: new
Abstract: The detection of suicide risk in social media is a critical task with potential life-saving implications. This paper presents a study on leveraging state-of-the-art natural language processing solutions for identifying suicide risk in social media posts as a submission for the “IEEE BigData 2024 Cup: Detection of Suicide Risk on Social Media” conducted by the kubapok team. We experimented with the following configurations of transformer-based models: fine-tuned DeBERTa, GPT-4o with CoT and few-shot prompting, and fine-tuned GPT-4o. The task setup was to classify social media posts into four categories: indicator, ideation, behavior, and attempt. Our findings demonstrate that the fine-tuned GPT-4o model outperforms two other configurations, achieving high accuracy in identifying suicide risk. Notably, our model achieved second place in the competition. By demonstrating that straightforward, general-purpose models can achieve state-of-the-art results, we propose that these models, combined with minimal tuning, may have the potential to be effective solutions for automated suicide risk detection on social media.
Source link
lol
Evaluating Transformer Models for Suicide Risk Detection on Social Media
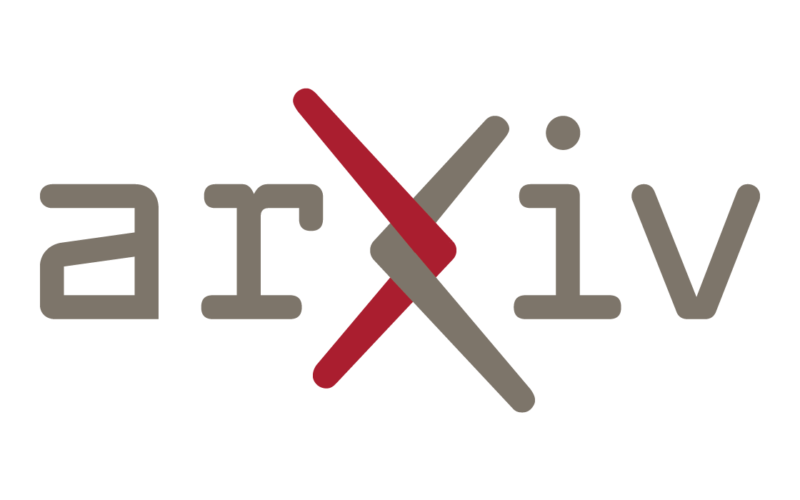