arXiv:2410.03812v1 Announce Type: new
Abstract: The advancement of dense visual simultaneous localization and mapping (SLAM) has been greatly facilitated by the emergence of neural implicit representations. Neural implicit encoding SLAM, a typical example of which is NICE-SLAM, has recently demonstrated promising results in large-scale indoor scenes. However, these methods typically rely on temporally dense RGB-D image streams as input in order to function properly. When the input source does not support high frame rates or the camera movement is too fast, these methods often experience crashes or significant degradation in tracking and mapping accuracy. In this paper, we propose EvenNICER-SLAM, a novel approach that addresses this issue through the incorporation of event cameras. Event cameras are bio-inspired cameras that respond to intensity changes instead of absolute brightness. Specifically, we integrated an event loss backpropagation stream into the NICE-SLAM pipeline to enhance camera tracking with insufficient RGB-D input. We found through quantitative evaluation that EvenNICER-SLAM, with an inclusion of higher-frequency event image input, significantly outperforms NICE-SLAM with reduced RGB-D input frequency. Our results suggest the potential for event cameras to improve the robustness of dense SLAM systems against fast camera motion in real-world scenarios.
Source link
lol
EvenNICER-SLAM: Event-based Neural Implicit Encoding SLAM
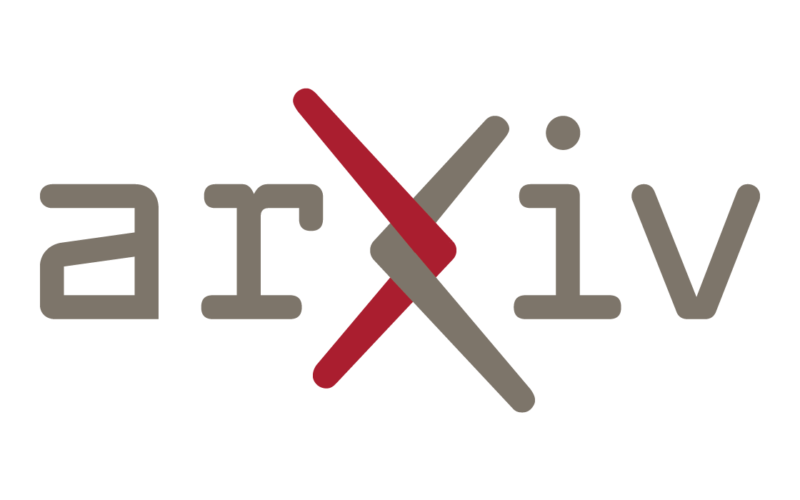