(Vitalii Stock/Shutterstock)
There are many causes of data corruption in our world, including human error, malware, and even cosmic rays. Now the folks at data observability firm Monte Carlo have come up with a way to detect another source of erroneous data: code changes in Databricks and pull requests in GitLab.
The folks at Monte Carlo live and breathe data quality, which has always been a problem but is getting more airtime today thanks to growing interest in AI. The company develops observability tools designed to help detect when the quality of data begins to drop as it makes its way through enterprise data pipelines.
Data transformation, or the “T” in the ETL, has always been a data engineering challenge. On the one hand, raw data typically must be modified in some way before it can be used for analytics or training machine learning models. On the other hand, the transformations can be quite complex, and detecting when something goes awry can be difficult.
With today’s announcement, Monte Carlo says it has developed root cause analysis (RCA) capabilities that allow customers detect when code changes in Databricks and GitLab environments cause the data quality to deteriorate in downstream systems.
According to Monte Carlo CTO and Co-founder Lior Gavish, the new RCA feature leverage new data that Databricks only started to share recently.
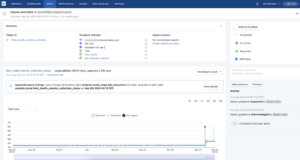
Monte Carlo provides automated root cause analysis capability for Databricks, Snowflake, GitHub, and GitLab (Image source: Monte Carlo)
“Up until this summer, Databricks did not allow vendors to access query logs directly,” Gavish tells BigDATAwire. “So when they made this functionality available, we jumped at the opportunity and built the integration quickly. With this new release, we can collect Databricks’ query history so we can surface information about Databricks’ query logs to our customers.”
The new features enable customers to detect when changes they have made in their Databricks environment is the root cause of the data quality failure. Users can also see how this query change impacted downstream dependencies and understand upstream correlations, Gavish says.
Monte Carlo has done similar work to identify when coding changes that customers maintain in their GitLab repositories is the root cause of downstream data quality issues.
“Now customers leveraging GitLab as their repository can leverage this new integration to get visibility into the impact of buggy source code or breaking pull requests on their downstream data assets,” Gavish says.
The RCA work Monte Carlo has done with Databricks and GitLab follow similar efforts with their two main rivals, Snowflake and GitHub. Gavish says that’s a result of the company’s efforts to “meet customers where they are, regardless of what their data estate looks like or how it’s evolving.”
“We’re always looking to take the next step in providing end-to-end detection and resolution across the data stack, whether those issues arise in your data, your systems, or your code,” he tells us. “To that end, we’ll continue to leverage query logs and repository source code for other data sources (i.e. SQL server, Microsoft Fabric, etc.) and other code repositories (ex: Azure devops).”
Gavish says that, based on the company’s experience in monitoring more than 10 million tables, about one-third of the data errors stem from code-related root causes. Once detected, it takes an average of 15 hours to resolve a data issue once an anomaly has been detected, the company says. The speedup provided by automated RCA detection can reduce the time to resolution by 80%, Gavish says.
Related Items:
Data Quality Is Getting Worse, Monte Carlo Says
Monte Carlo Raises $135 Million to Grow Data Observability Biz
Source link
lol