arXiv:2409.18205v1 Announce Type: new
Abstract: In the context of modern machine learning, models deployed in real-world scenarios often encounter diverse data shifts like covariate and semantic shifts, leading to challenges in both out-of-distribution (OOD) generalization and detection. Despite considerable attention to these issues separately, a unified framework for theoretical understanding and practical usage is lacking. To bridge the gap, we introduce a graph-theoretic framework to jointly tackle both OOD generalization and detection problems. By leveraging the graph formulation, data representations are obtained through the factorization of the graph’s adjacency matrix, enabling us to derive provable error quantifying OOD generalization and detection performance. Empirical results showcase competitive performance in comparison to existing methods, thereby validating our theoretical underpinnings. Code is publicly available at https://github.com/deeplearning-wisc/graph-spectral-ood.
Source link
lol
Bridging OOD Detection and Generalization: A Graph-Theoretic View
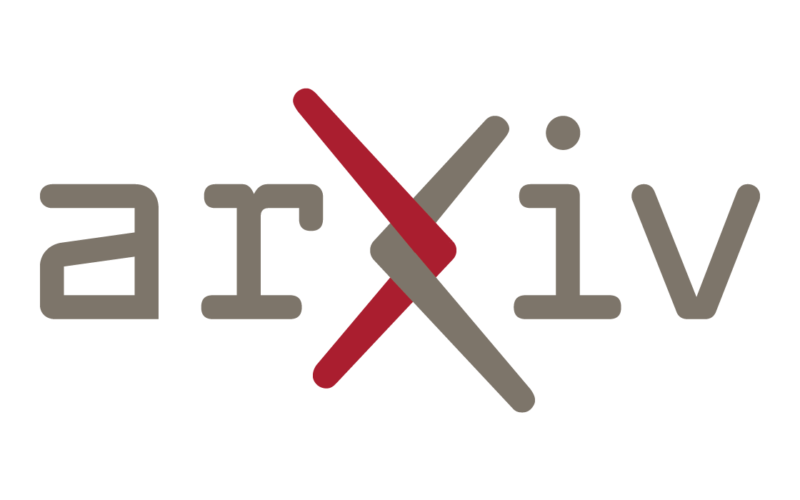