arXiv:2409.18260v1 Announce Type: new
Abstract: For automatic human figure drawing (HFD) assessment tasks, such as diagnosing autism spectrum disorder (ASD) using HFD images, the clarity and explainability of a model decision are crucial. Existing pixel-level attribution-based explainable AI (XAI) approaches demand considerable effort from users to interpret the semantic information of a region in an image, which can be often time-consuming and impractical. To overcome this challenge, we propose a part contribution evaluation based model explanation (PCEvE) framework. On top of the part detection, we measure the Shapley Value of each individual part to evaluate the contribution to a model decision. Unlike existing attribution-based XAI approaches, the PCEvE provides a straightforward explanation of a model decision, i.e., a part contribution histogram. Furthermore, the PCEvE expands the scope of explanations beyond the conventional sample-level to include class-level and task-level insights, offering a richer, more comprehensive understanding of model behavior. We rigorously validate the PCEvE via extensive experiments on multiple HFD assessment datasets. Also, we sanity-check the proposed method with a set of controlled experiments. Additionally, we demonstrate the versatility and applicability of our method to other domains by applying it to a photo-realistic dataset, the Stanford Cars.
Source link
lol
PCEvE: Part Contribution Evaluation Based Model Explanation for Human Figure Drawing Assessment and Beyond
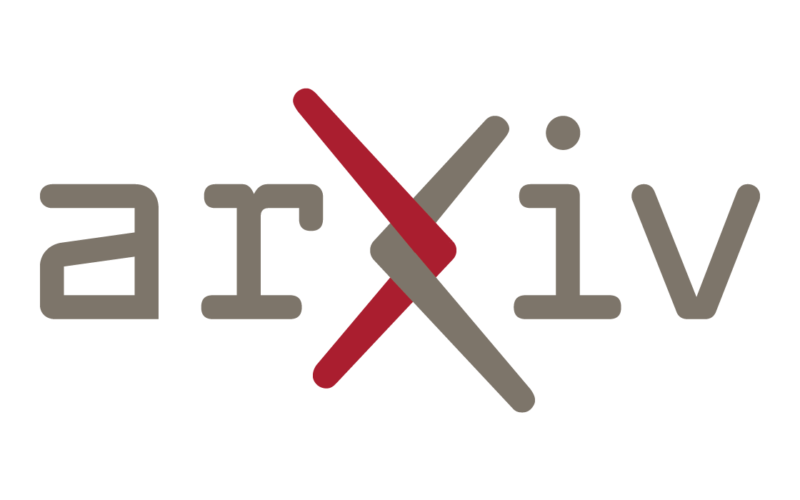