arXiv:2409.17730v1 Announce Type: cross
Abstract: The goal of modern sequential recommender systems is often formulated in terms of next-item prediction. In this paper, we explore the applicability of generative transformer-based models for the Top-K sequential recommendation task, where the goal is to predict items a user is likely to interact with in the “near future”.
We explore commonly used autoregressive generation strategies, including greedy decoding, beam search, and temperature sampling, to evaluate their performance for the Top-K sequential recommendation task. In addition, we propose novel Reciprocal Rank Aggregation (RRA) and Relevance Aggregation (RA) generation strategies based on multi-sequence generation with temperature sampling and subsequent aggregation.
Experiments on diverse datasets give valuable insights regarding commonly used strategies’ applicability and show that suggested approaches improve performance on longer time horizons compared to widely-used Top-K prediction approach and single-sequence autoregressive generation strategies.
Source link
lol
Autoregressive Generation Strategies for Top-K Sequential Recommendations
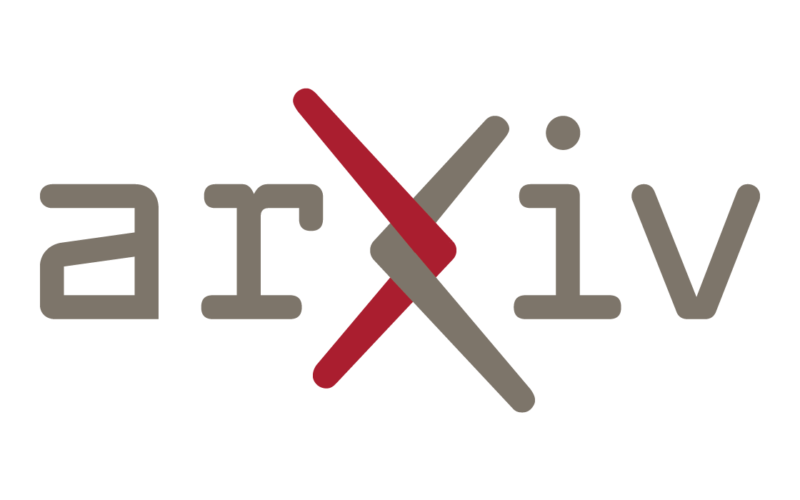