arXiv:2409.15331v1 Announce Type: new
Abstract: The utility of Synthetic Aperture Radar (SAR) imagery in remote sensing and satellite image analysis is well established, offering robustness under various weather and lighting conditions. However, SAR images, characterized by their unique structural and texture characteristics, often pose interpretability challenges for analysts accustomed to electrooptical (EO) imagery. This application compares state-of-the-art Generative Adversarial Networks (GANs) including Pix2Pix, CycleGan, S-CycleGan, and a novel dual?generator GAN utilizing partial convolutions and a novel dual-generator architecture utilizing transformers. These models are designed to progressively refine the realism in the translated optical images, thereby enhancing the visual interpretability of SAR data. We demonstrate the efficacy of our approach through qualitative and quantitative evaluations, comparing the synthesized EO images with actual EO images in terms of visual fidelity and feature preservation. The results show significant improvements in interpretability, making SAR data more accessible for analysts familiar with EO imagery. Furthermore, we explore the potential of this technology in various applications, including environmental monitoring, urban planning, and military reconnaissance, where rapid, accurate interpretation of SAR data is crucial. Our research contributes to the field of remote sensing by bridging the gap between SAR and EO imagery, offering a novel tool for enhanced data interpretation and broader application of SAR technology in various domains.
Source link
lol
Electrooptical Image Synthesis from SAR Imagery Using Generative Adversarial Networks
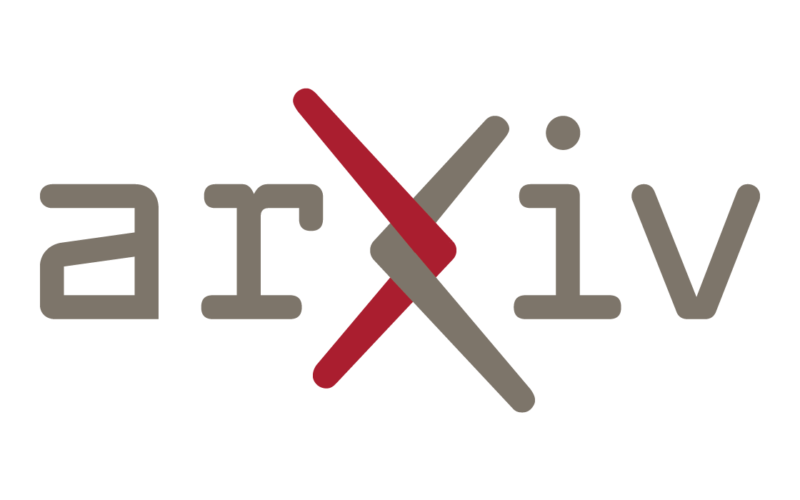