arXiv:2409.11635v1 Announce Type: new
Abstract: Pain is a more intuitive and user-friendly way of communicating problems, making it especially useful in rehabilitation nurse training robots. While most previous methods have focused on classifying or recognizing pain expressions, these approaches often result in unnatural, jiggling robot faces. We introduce PainDiffusion, a model that generates facial expressions in response to pain stimuli, with controllable pain expressiveness and emotion status. PainDiffusion leverages diffusion forcing to roll out predictions over arbitrary lengths using a conditioned temporal U-Net. It operates as a latent diffusion model within EMOCA’s facial expression latent space, ensuring a compact data representation and quick rendering time. For training data, we process the BioVid Heatpain Database, extracting expression codes and subject identity configurations. We also propose a novel set of metrics to evaluate pain expressions, focusing on expressiveness, diversity, and the appropriateness of model-generated outputs. Finally, we demonstrate that PainDiffusion outperforms the autoregressive method, both qualitatively and quantitatively. Code, videos, and further analysis are available at: href{https://damtien444.github.io/paindf/}{https://damtien444.github.io/paindf/}.
Source link
lol
PainDiffusion: Can robot express pain?
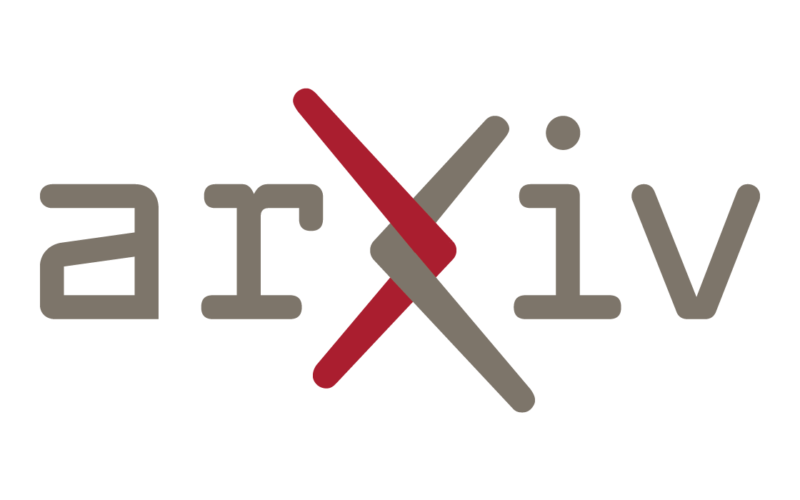