arXiv:2409.11350v1 Announce Type: cross
Abstract: Machine-learning (ML) models in flow cytometry have the potential to reduce error rates, increase reproducibility, and boost the efficiency of clinical labs. While numerous ML models for flow cytometry data have been proposed, few studies have described the clinical deployment of such models. Realizing the potential gains of ML models in clinical labs requires not only an accurate model, but infrastructure for automated inference, error detection, analytics and monitoring, and structured data extraction. Here, we describe an ML model for detection of Acute Myeloid Leukemia (AML), along with the infrastructure supporting clinical implementation. Our infrastructure leverages the resilience and scalability of the cloud for model inference, a Kubernetes-based workflow system that provides model reproducibility and resource management, and a system for extracting structured diagnoses from full-text reports. We also describe our model monitoring and visualization platform, an essential element for ensuring continued model accuracy. Finally, we present a post-deployment analysis of impacts on turn-around time and compare production accuracy to the original validation statistics.
Source link
lol
Clinical Validation of a Real-Time Machine Learning-based System for the Detection of Acute Myeloid Leukemia by Flow Cytometry
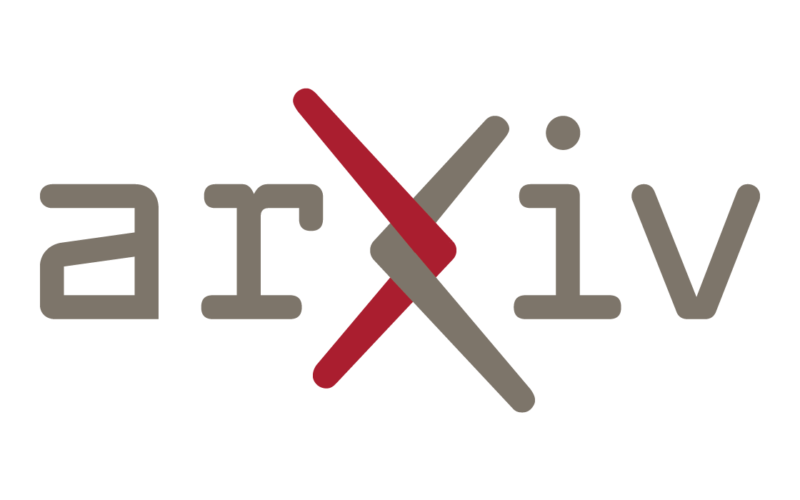