arXiv:2409.08482v1 Announce Type: new
Abstract: With the emerging trend in generative models and convenient public access to diffusion models pre-trained on large datasets, users can fine-tune these models to generate images of personal faces or items in new contexts described by natural language. Parameter efficient fine-tuning (PEFT) such as Low Rank Adaptation (LoRA) has become the most common way to save memory and computation usage on the user end during fine-tuning. However, a natural question is whether the private images used for fine-tuning will be leaked to adversaries when sharing model weights. In this paper, we study the issue of privacy leakage of a fine-tuned diffusion model in a practical setting, where adversaries only have access to model weights, rather than prompts or images used for fine-tuning. We design and build a variational network autoencoder that takes model weights as input and outputs the reconstruction of private images. To improve the efficiency of training such an autoencoder, we propose a training paradigm with the help of timestep embedding. The results give a surprising answer to this research question: an adversary can generate images containing the same identities as the private images. Furthermore, we demonstrate that no existing defense method, including differential privacy-based methods, can preserve the privacy of private data used for fine-tuning a diffusion model without compromising the utility of a fine-tuned model.
Source link
lol
Risks When Sharing LoRA Fine-Tuned Diffusion Model Weights
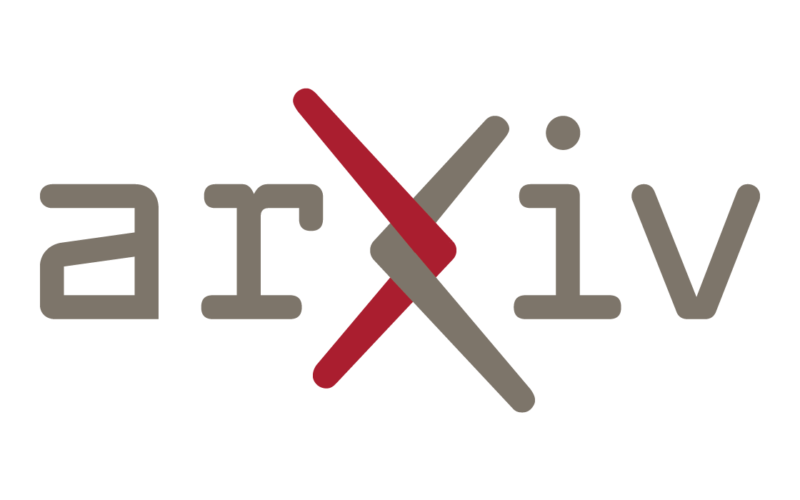