arXiv:2406.00258v1 Announce Type: new
Abstract: Videos carry rich visual information including object description, action, interaction, etc., but the existing multimodal large language models (MLLMs) fell short in referential understanding scenarios such as video-based referring. In this paper, we present Artemis, an MLLM that pushes video-based referential understanding to a finer level. Given a video, Artemis receives a natural-language question with a bounding box in any video frame and describes the referred target in the entire video. The key to achieving this goal lies in extracting compact, target-specific video features, where we set a solid baseline by tracking and selecting spatiotemporal features from the video. We train Artemis on the newly established VideoRef45K dataset with 45K video-QA pairs and design a computationally efficient, three-stage training procedure. Results are promising both quantitatively and qualitatively. Additionally, we show that model can be integrated with video grounding and text summarization tools to understand more complex scenarios. Code and data are available at https://github.com/qiujihao19/Artemis.
Source link
lol
Artemis: Towards Referential Understanding in Complex Videos
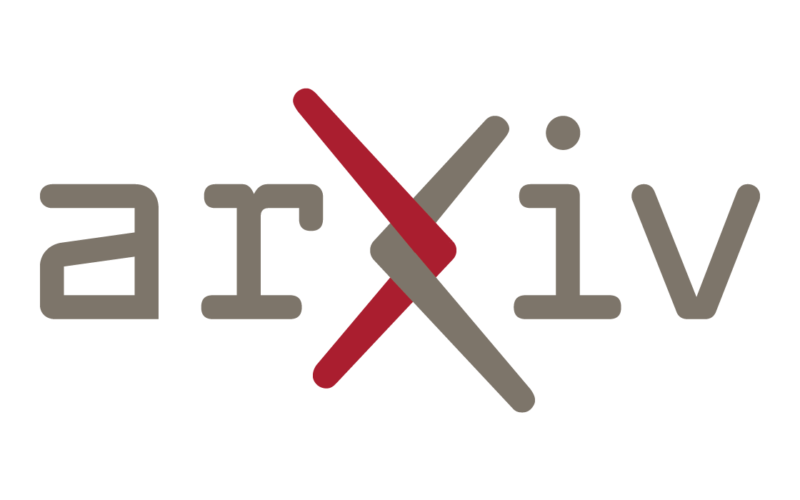