arXiv:2409.07606v1 Announce Type: new
Abstract: Deep learning regularization techniques, such as emph{dropout}, emph{layer normalization}, or emph{weight decay}, are widely adopted in the construction of modern artificial neural networks, often resulting in more robust training processes and improved generalization capabilities. However, in the domain of emph{Reinforcement Learning} (RL), the application of these techniques has been limited, usually applied to value function estimators citep{hiraoka2021dropout, smith2022walk}, and may result in detrimental effects. This issue is even more pronounced in offline RL settings, which bear greater similarity to supervised learning but have received less attention. Recent work in continuous offline RL has demonstrated that while we can build sufficiently powerful critic networks, the generalization of actor networks remains a bottleneck. In this study, we empirically show that applying standard regularization techniques to actor networks in offline RL actor-critic algorithms yields improvements of 6% on average across two algorithms and three different continuous D4RL domains.
Source link
lol
The Role of Deep Learning Regularizations on Actors in Offline RL
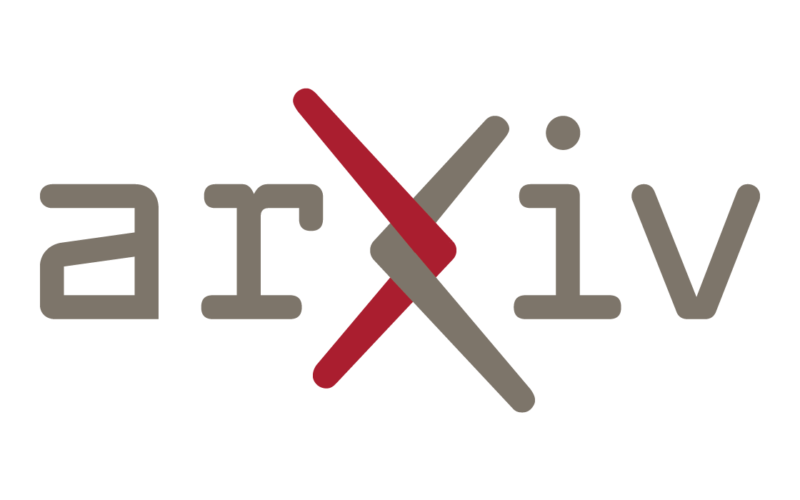