arXiv:2406.00020v1 Announce Type: new
Abstract: Content moderation on social media platforms shapes the dynamics of online discourse, influencing whose voices are amplified and whose are suppressed. Recent studies have raised concerns about the fairness of content moderation practices, particularly for aggressively flagging posts from transgender and non-binary individuals as toxic. In this study, we investigate the presence of bias in harmful speech classification of gender-queer dialect online, focusing specifically on the treatment of reclaimed slurs. We introduce a novel dataset, QueerReclaimLex, based on 109 curated templates exemplifying non-derogatory uses of LGBTQ+ slurs. Dataset instances are scored by gender-queer annotators for potential harm depending on additional context about speaker identity. We systematically evaluate the performance of five off-the-shelf language models in assessing the harm of these texts and explore the effectiveness of chain-of-thought prompting to teach large language models (LLMs) to leverage author identity context. We reveal a tendency for these models to inaccurately flag texts authored by gender-queer individuals as harmful. Strikingly, across all LLMs the performance is poorest for texts that show signs of being written by individuals targeted by the featured slur (F1 <= 0.24). We highlight an urgent need for fairness and inclusivity in content moderation systems. By uncovering these biases, this work aims to inform the development of more equitable content moderation practices and contribute to the creation of inclusive online spaces for all users.
Source link
lol
Harmful Speech Detection by Language Models Exhibits Gender-Queer Dialect Bias
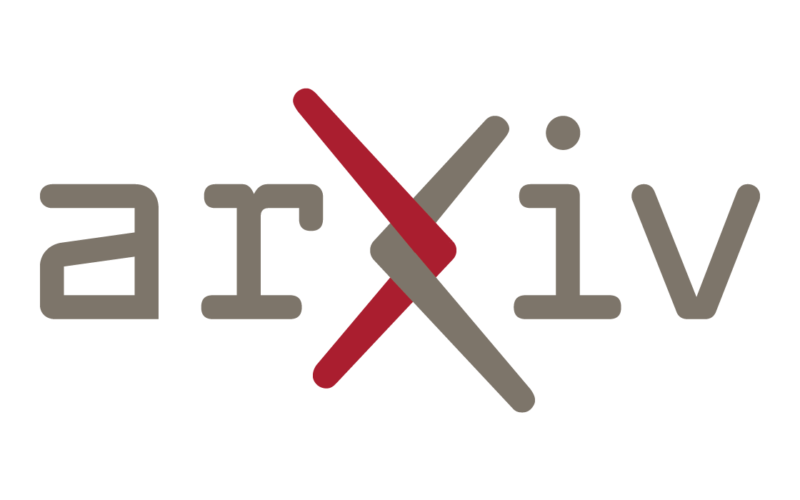