arXiv:2408.12638v1 Announce Type: new
Abstract: Fault detection in automotive engine systems is one of the most promising research areas. Several works have been done in the field of model-based fault diagnosis. Many researchers have discovered more advanced statistical methods and algorithms for better fault detection on any automotive dynamic engine system. The gas turbines/diesel engines produce highly complex and huge data which are highly non-linear. So, researchers should come up with an automated system that is more resilient and robust enough to handle this huge, complex data in highly non-linear dynamic automotive systems. Here, I present an AI-based fault classification and prediction model in the diesel engine that can be applied to any highly non-linear dynamic automotive system. The main contribution of this paper is the AI-based Transformer fault classification and prediction model in the diesel engine concerning the worldwide harmonic light vehicle test procedure (WLTP) driving cycle. This model used 27 input dimensions, 64 hidden dimensions with 2 layers, and 9 heads to create a classifier with 12 output heads (one for fault-free data and 11 different fault types). This model was trained on the UTSA Arc High-Performance Compute (HPC) cluster with 5 NVIDIA V100 GPUs, 40-core CPUs, and 384GB RAM and achieved 70.01 % accuracy on a held test set.
Source link
lol
AI-driven Transformer Model for Fault Prediction in Non-Linear Dynamic Automotive System
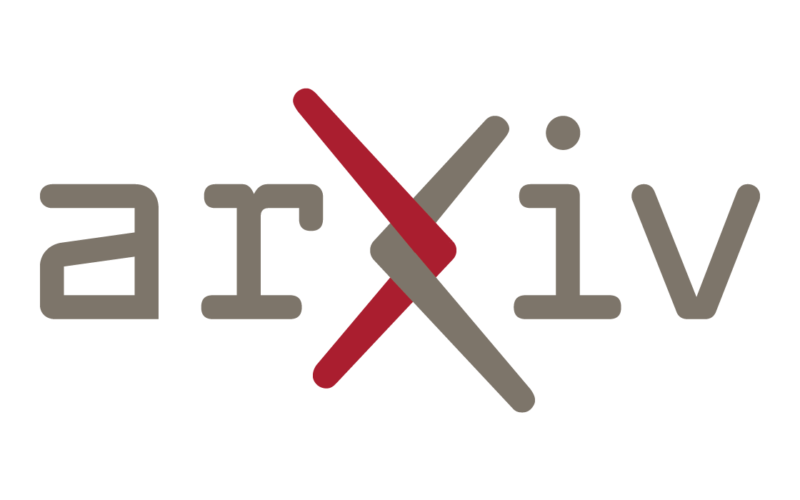