arXiv:2408.11843v1 Announce Type: new
Abstract: Generating fair and accurate predictions plays a pivotal role in deploying large language models (LLMs) in the real world. However, existing debiasing methods inevitably generate unfair or incorrect predictions as they are designed and evaluated to achieve parity across different social groups but leave aside individual commonsense facts, resulting in modified knowledge that elicits unreasonable or undesired predictions. In this paper, we first establish a new bias mitigation benchmark, BiaScope, which systematically assesses performance by leveraging newly constructed datasets and metrics on knowledge retention and generalization. Then, we propose a novel debiasing approach, Fairness Stamp (FAST), which enables fine-grained calibration of individual social biases. FAST identifies the decisive layer responsible for storing social biases and then calibrates its outputs by integrating a small modular network, considering both bias mitigation and knowledge-preserving demands. Comprehensive experiments demonstrate that FAST surpasses state-of-the-art baselines with superior debiasing performance while not compromising the overall model capability for knowledge retention and downstream predictions. This highlights the potential of fine-grained debiasing strategies to achieve fairness in LLMs. Code will be publicly available.
Source link
lol
Editable Fairness: Fine-Grained Bias Mitigation in Language Models
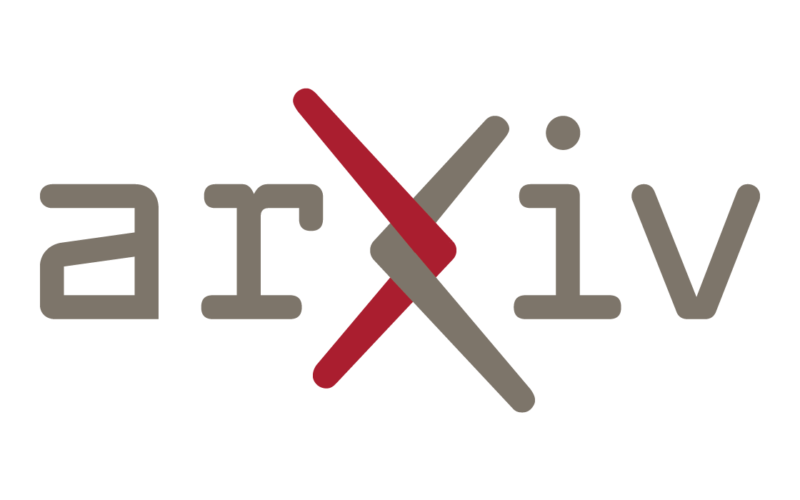