arXiv:2408.07962v1 Announce Type: new
Abstract: Safe Reinforcement Learning (Safe RL) is one of the prevalently studied subcategories of trial-and-error-based methods with the intention to be deployed on real-world systems. In safe RL, the goal is to maximize reward performance while minimizing constraints, often achieved by setting bounds on constraint functions and utilizing the Lagrangian method. However, deploying Lagrangian-based safe RL in real-world scenarios is challenging due to the necessity of threshold fine-tuning, as imprecise adjustments may lead to suboptimal policy convergence. To mitigate this challenge, we propose a unified Lagrangian-based model-free architecture called Meta Soft Actor-Critic Lagrangian (Meta SAC-Lag). Meta SAC-Lag uses meta-gradient optimization to automatically update the safety-related hyperparameters. The proposed method is designed to address safe exploration and threshold adjustment with minimal hyperparameter tuning requirement. In our pipeline, the inner parameters are updated through the conventional formulation and the hyperparameters are adjusted using the meta-objectives which are defined based on the updated parameters. Our results show that the agent can reliably adjust the safety performance due to the relatively fast convergence rate of the safety threshold. We evaluate the performance of Meta SAC-Lag in five simulated environments against Lagrangian baselines, and the results demonstrate its capability to create synergy between parameters, yielding better or competitive results. Furthermore, we conduct a real-world experiment involving a robotic arm tasked with pouring coffee into a cup without spillage. Meta SAC-Lag is successfully trained to execute the task, while minimizing effort constraints.
Source link
lol
Meta SAC-Lag: Towards Deployable Safe Reinforcement Learning via MetaGradient-based Hyperparameter Tuning
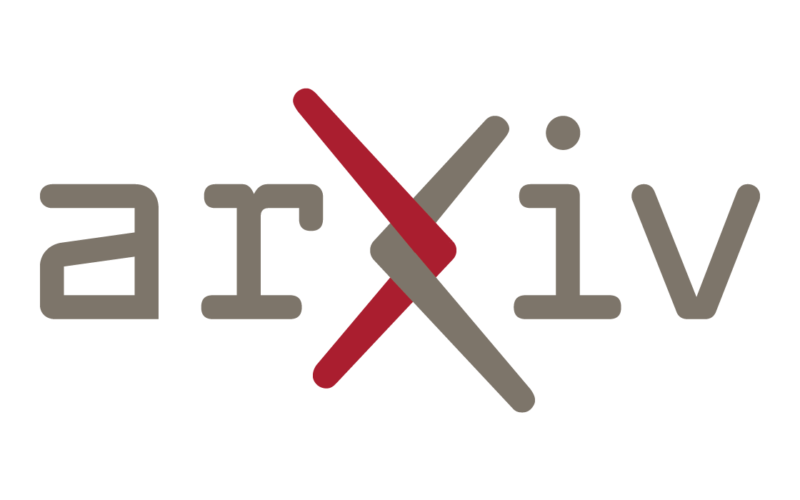