arXiv:2408.07925v1 Announce Type: new
Abstract: Sleep plays a crucial role in neonatal development. Monitoring the sleep patterns in neonates in a Neonatal Intensive Care Unit (NICU) is imperative for understanding the maturation process. While polysomnography (PSG) is considered the best practice for sleep classification, its expense and reliance on human annotation pose challenges. Existing research often relies on multichannel EEG signals; however, concerns arise regarding the vulnerability of neonates and the potential impact on their sleep quality. This paper introduces a novel approach to neonatal sleep stage classification using a single-channel gradient boosting algorithm with Hjorth features. The gradient boosting parameters are fine-tuned using random search cross-validation (randomsearchCV), achieving an accuracy of 82.35% for neonatal sleep-wake classification. Validation is conducted through 5-fold cross-validation. The proposed algorithm not only enhances existing neonatal sleep algorithms but also opens avenues for broader applications.
Source link
lol
A Single Channel-Based Neonatal Sleep-Wake Classification using Hjorth Parameters and Improved Gradient Boosting
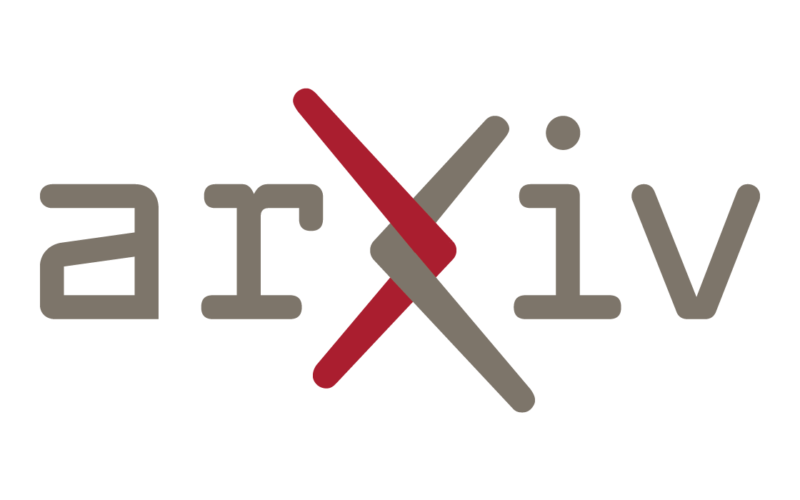