arXiv:2408.07989v1 Announce Type: new
Abstract: Knowledge-based visual question answering requires external knowledge beyond visible content to answer the question correctly. One limitation of existing methods is that they focus more on modeling the inter-modal and intra-modal correlations, which entangles complex multimodal clues by implicit embeddings and lacks interpretability and generalization ability. The key challenge to solve the above problem is to separate the information and process it separately at the functional level. By reusing each processing unit, the generalization ability of the model to deal with different data can be increased. In this paper, we propose Independent Inference Units (IIU) for fine-grained multi-modal reasoning to decompose intra-modal information by the functionally independent units. Specifically, IIU processes each semantic-specific intra-modal clue by an independent inference unit, which also collects complementary information by communication from different units. To further reduce the impact of redundant information, we propose a memory update module to maintain semantic-relevant memory along with the reasoning process gradually. In comparison with existing non-pretrained multi-modal reasoning models on standard datasets, our model achieves a new state-of-the-art, enhancing performance by 3%, and surpassing basic pretrained multi-modal models. The experimental results show that our IIU model is effective in disentangling intra-modal clues as well as reasoning units to provide explainable reasoning evidence. Our code is available at https://github.com/Lilidamowang/IIU.
Source link
lol
IIU: Independent Inference Units for Knowledge-based Visual Question Answering
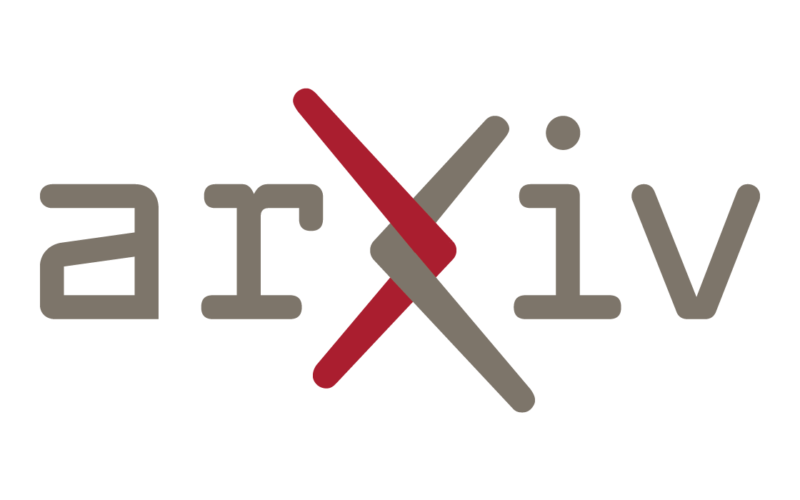