arXiv:2408.07884v1 Announce Type: new
Abstract: Abstract. Automatically generating scientific literature surveys is a valuable task that can significantly enhance research efficiency. However, the diverse and complex nature of information within a literature survey poses substantial challenges for generative models. In this paper, we design a series of prompts to systematically leverage large language models (LLMs), enabling the creation of comprehensive literature surveys through a step-by-step approach. Specifically, we design prompts to guide LLMs to sequentially generate the title, abstract, hierarchical headings, and the main content of the literature survey. We argue that this design enables the generation of the headings from a high-level perspective. During the content generation process, this design effectively harnesses relevant information while minimizing costs by restricting the length of both input and output content in LLM queries. Our implementation with Qwen-long achieved third place in the NLPCC 2024 Scientific Literature Survey Generation evaluation task, with an overall score only 0.03% lower than the second-place team. Additionally, our soft heading recall is 95.84%, the second best among the submissions. Thanks to the efficient prompt design and the low cost of the Qwen-long API, our method reduces the expense for generating each literature survey to 0.1 RMB, enhancing the practical value of our method.
Source link
lol
Instruct Large Language Models to Generate Scientific Literature Survey Step by Step
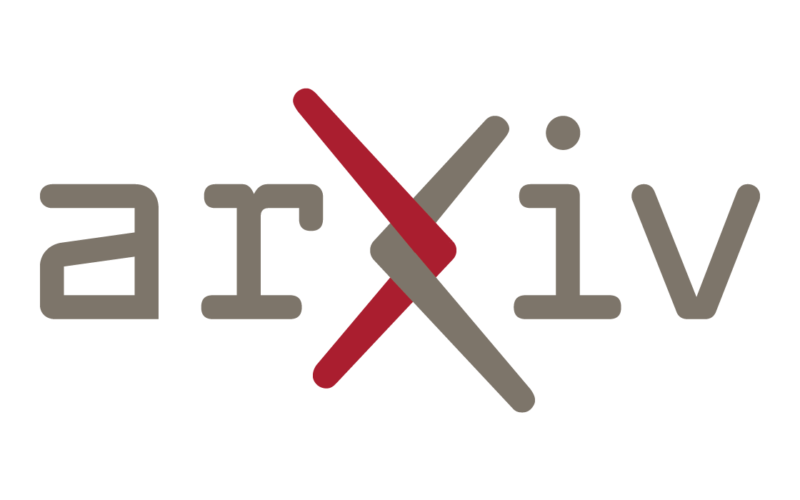