Snowflake Inc. is at a crossroads.
The company, which defined the modern data platform, now faces fresh challenges on four fronts. Specifically, Snowflake must: 1) advance its data cloud vision; 2) transform into an artificial intelligence leader; 3) become a platform for data apps; and 4) address external market forces that pressure its core values of simplicity, efficiency and trusted data.
Our premise is that open storage formats, a shift toward data catalogs governing and defining data, and the AI awakening will challenge Snowflake’s ability to extend its value proposition, create novel moats and compete in new markets. Although Snowflake has historically thrived against hyperscale competition with a superior value story in a market it created, the redefinition of the modern data platform puts it in a more challenging position. We believe the outcome will depend on how well it integrates generative AI into its products and how quickly it can innovate relative to competitors.
Specifically, though Snowflake has the strongest engine, competitors specializing in tools for business analysts, data scientists, data analysts and end users may be better positioned to add gen AI. To compete directly, Snowflake must leverage its engine and metadata to create a unique, simplified gen AI experience that other tools cannot match. To succeed in its new ambitions, the company must flawlessly execute on both organic and inorganic innovation while attracting new personas, building an ecosystem of developers, and balancing profit margins as a public entity.
In this Breaking Analysis, we’ll share how we see the next data platform – that is, the sixth data platform – evolving. And we’ll give you a preview of what we expect at next week’s Snowflake Data Cloud Summit in the context of our premise.
Snowflake defined the fifth data platform. What’s next?
Snowflake earned its leadership position with a superior product. Its visionary founders recognized that the cloud enabled the separation of compute from storage, offering virtually infinite scalability on demand. By building an integrated data platform with comprehensive governance, Snowflake maintained tight control over data and became a trusted source of analytical data. This well-integrated system made it easy for partners to add value in areas such as data pipelines and data quality, allowing the company to pioneer efficient and trusted data sharing across ecosystems.
The company built its stack (depicted above left) on top of cloud storage and developed an extremely efficient compute engine in the form of a modern cloud database with built-in governance from Snowflake and its ecosystem partners. As it evolved beyond the notion of a cloud data warehouse into what the company calls a data cloud, a next logical total available market expansion strategy was to become the app store for data applications.
Several forces have combined to change the game. The AI awakening has accelerated demand for new ways of interacting with data and competitive pressure has created demand for open data formats such as Iceberg. No longer does it suffice to separate compute from storage, rather customers want to apply any compute to any data in an open read/write model that is governed. This demand for openness has shifted the data platform moat toward catalogs that are becoming the new point of control.
No longer does it suffice to separate compute from storage. Rather, customers want to apply any compute to any data in an open read/write model that is governed.
Last June, Databricks Inc.announced its Unity Catalog. Databricks never “owned” the data the way Snowflake does. It had nothing to lose, so it changed the game with Unity, which includes both technical and governance metadata and essentially shifts the point of control in an open format.
Snowflake, the integration of the modern data stack, and the simplicity of the walled garden
As we’ve indicated, Snowflake defined the modern data stack where compute and storage were separate but came from a single vendor. That separation delivered basically infinite scalability of each resource.
But any workloads that customers wanted to run on their data had to go through Snowflake’s data management compute engine. In a way, it was a walled garden. When customers wanted Python programmability for data science work, Snowflake added Snowpark. Ultimately, Snowflake Container Services allowed any container-based compute engine to work with Snowflake data – as long as it accessed the Snowflake data management compute engine.
This idea of data ownership is fundamental to Snowflake’s business model. Any customer wanting to use any third-party tool on Snowflake data had to go through Snowflake’s engine. And Snowflake meters consumption of any access to the data through their engine. So this architecture was also fundamental to Snowflake’s monetization model.
Interoperability of open data storage and the catalog as a new point of control
The promise of the Iceberg table format specifically and open table formats in general is that no one vendor’s compute engine “owns” the data. Customers can use many tools and many engines for specialized workloads and applications. In order to manage a separation of compute and data, the source of truth and the point of control shifts from the database management system-based compute engine to the catalog that defines and governs the data. That catalog has to be connected to the open table storage engine so that it can mediate all compute engines.
Databricks always had open data storage and less advanced DBMS technology than Snowflake. So it’s no surprise that it was the first to push the frontier of a metadata catalog that aspired to manage an entire open analytic data estate at its conference last year.
In order to manage a separation of compute and data, the source of truth and the point of control shifts from the DBMS-based compute engine to the catalog that defines and governs the data.
Snowflake’s challenge: separating metadata governance from data management
The question is how Snowflake will make this transition, both technically and commercially. Its metadata catalog and governance has always been bundled into the DBMS compute engine. The simplicity of Snowflake’s native tools has always rested on the capability and extensibility of its powerful data management engine combined with its governance metadata. How it lays out a path for separating these will be critical.
Double-clicking on this mandate, the graphic below adds additional color by adding Iceberg and native tables in the mix.
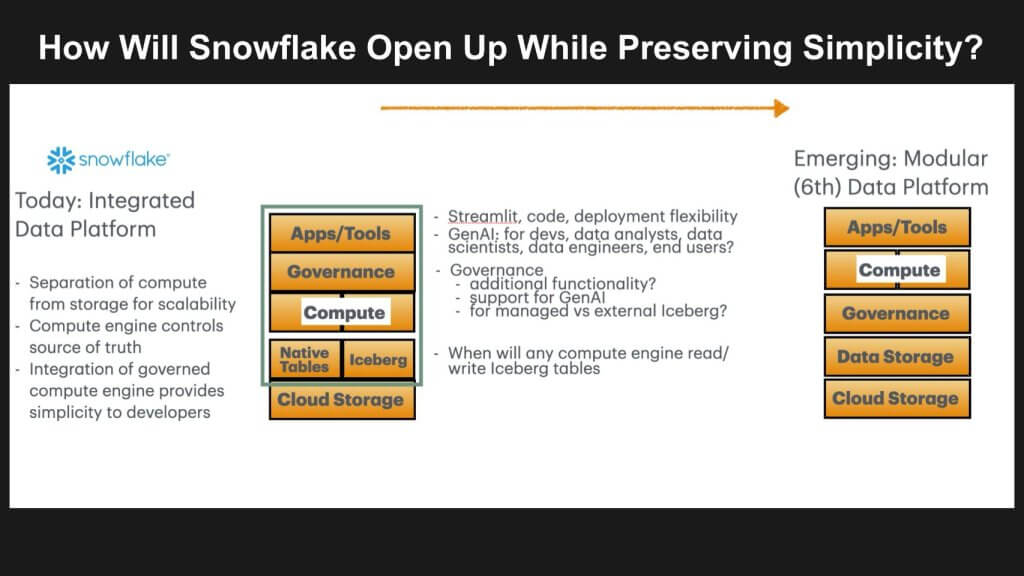
A key challenge for Snowflake as it responds to demands for open table formats is that it must extend its value proposition to those open data tables. To do so, however, risks handing off its moat to the broader industry. At the same time, it could bring Iceberg tables into its platform and make Iceberg a first-class citizen with full integration, governance and read:write access. But doing so runs somewhat counter to the trends toward openness.
The vision of the sixth data platform as we call it is that any compute engine can read from and update to open tables. Rather than simply separating compute from storage, which defined the fifth data platform, the sixth data platform, we believe, will allow any compute full access to any data.
Rather than simply separating compute from storage, which defined the 5th data platform, the 6th data platform we believe will allow any compute full access to any data.
Snowflake announced its intent to support Iceberg tables at its Summit 2022. But like passengers on the Titanic, not all Iceberg tables are first-class citizens. Managed Iceberg tables, announced in preview at last year’s summit, get all the rich governance and metadata that enables them to plug into Snowflake’s full functionality with simplicity and performance. The advantage is that third-party tools can read Managed Iceberg tables without going through Snowflake.
External Iceberg tables are essentially outside the data platform. Snowflake can’t write to them. Customers are responsible for governance. That means another technical metadata catalog has to serve as the source of truth about the state of the data. Third party tools can read and write these tables. But they lose the rich Snowflake governance and metadata that makes Managed Iceberg tables a seamlessly simple part of Snowflake workloads. In other words, External Iceberg tables are second-class citizens.
So the big question is… if, when, and how all Iceberg tables become first-class citizens both within Snowflake and externally. That will require moving the governance and metadata from something integrated with the DBMS compute engine to something integrated with the Iceberg data. That’s not easy technically and it presents friction commercially.
Gen AI creates new demand for working with all types of data
Sridhar Ramaswamy, Snowflake’s new chief executive, has talked publicly and emphatically about the need to integrate gen AI throughout the product. He has been elevated to an “AI wartime leader,” to quote our colleague John Furrier, and has made recent acquisitions such as TruEra to address the quality of machine learning and AI models. Cortex, Snowflake’s AI managed service announced this past winter, is another great example of how Snowflake is evolving into an AI company providing large language models as seamless, serverless extensions of SQL and Snowpark programmability.
But as Benoit Dageville, Snowflake’s visionary co-founder, shared with theCUBE Research this week, Snowflake wants to go further. It has always wanted to appeal to every user in an organization. That means it wants to reach end-users (for example, business users) who don’t normally interact with Snowflake. Dageville’s vision is for Snowflake to become the iPhone of data apps. This is both a great opportunity and a challenging aspiration.
Data interoperability requires a semantic layer. Is Snowflake iPhone or Android?
When Matei Zaharia, the creator of Spark and co-founder of Databricks, announced the Unity Catalog and the company’s gen AI strategy last year, he singled out the problem of company-specific language or “semantics.” It’s really hard for an LLM to translate the semantics of business language for each company into the table and column names of thousands or even hundreds of thousands of tables. We agree.
A wide spectrum of approaches exist to solve this problem, starting with the semantic layers of business intelligence tools such as Looker’s LookML to AtScale to dbt Labs’ semantic layer. At the other end of the spectrum, there are executable knowledge graphs built on a completely different database, such as EnterpriseWeb and RelationalAI.
Our interpretation of Snowflake’s position in this regard is that generalizing semantics as we’ve described them here is a risky undertaking that takes Snowflake off its core focus. In other words, we believe that Snowflake believes managing semantics is best left to application developers.
TheCUBE Research feels, however, that Snowflake has to put some stake in the ground here. Analytic data estates exist primarily to integrate and harmonize data from operational applications with their siloed semantics. Without this technology at the core, gen AI technology from other vendors that explicitly manage semantics for Snowflake queries may perform better on Snowflake data than Snowflake’s own gen AI query tools.
Why is this a problem? If Snowflake can be the platform and meter its usage, won’t it still get paid? Our view is yes, but this breaks the seamless interoperability across its application ecosystem. For example, if a third-party copilot builds on third-party semantics and performs better than Snowflake’s offerings, a bevy of third-party tools will be adopted and mixing and matching data across applications becomes more difficult. Stretching the analogy, Snowflake would be more Android-like than creating a seamless iPhone experience for data interoperability.
We need to better understand how Snowflake will address this challenge and to what degree the company can maintain its vision of iPhone integration and simplicity throughout the stack.
Snowflake thrived in a world of hyperscale competition. Here’s the next territorial advance: a Salesforce example
We joked up front that Snowflake is jumping from the frying pan into the fire. Below is an example of what we mean using Salesforce Inc.’s Data Cloud as a reference.
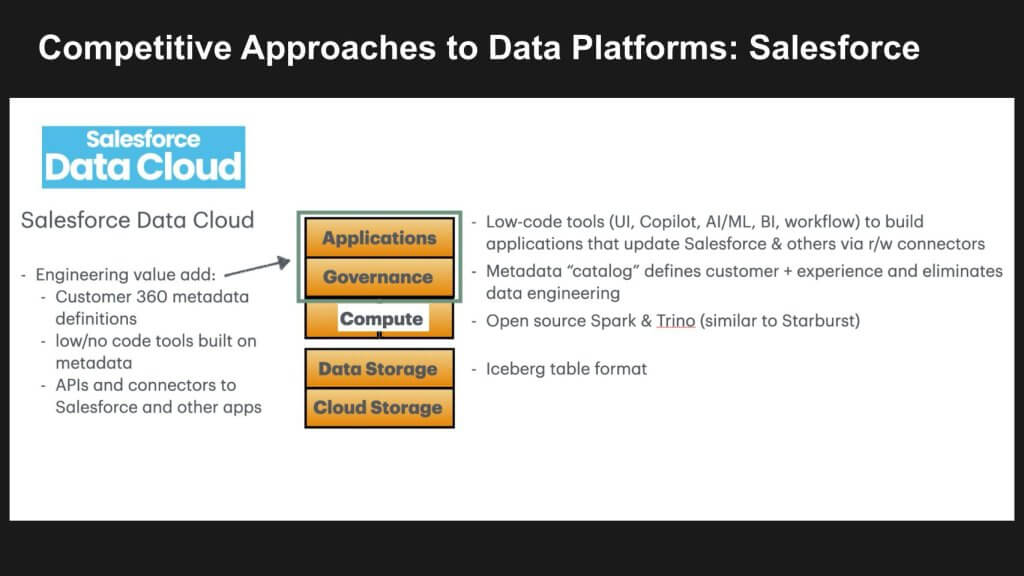
It’s interesting to note how that term data cloud has caught on. Snowflake first used it, Google uses it and so does Salesforce. The Salesforce Data Cloud is a data management platform designed to unify customer data from various sources, so that organizations can create a single view of their customer data.
Within Salesforce, business objects are predefined so all the coding for data engineering becomes a simple exercise in no-/low-code configurations. Salesforce then adds value on top. This approach has two main benefits: 1) It simplifies the building of applications with “out-of-the-box” low code user interfaces, intelligent copilots, machine intelligence, visualization and data workflows; and 2) These apps operationalize the decisions that the analytics inform by integrating with the core Salesforce apps and other applications quite easily. All their value-add is above the DBMS compute and data storage layer.
The point is, Salesforce has the data definitions for Customer 360 built right in, Snowflake does not. Is this is a gap Snowflake must fill? If so, how will it do so?
Snowflake could add value here and it relates to the issue of data semantics we raised in the previous section with respect to supporting gen AI. Data platforms exist to harmonize the data from different operational applications, each of which has its own semantics. Two potential solutions include: 1) Snowflake could provide semantic modeling and master data management tools; and 2) it could ship with extensible data models for customer 360 or operations 360 that work as starter kits.
Salesforce differentiation in this space is that it has the Customer 360 semantics, deep integration with operational apps and ML models that can learn from the experience of what happens in the operational apps. The simplicity that Snowflake aspires to will ultimately require better semantic modeling that is consistent and becomes an extension of its core engine. In this regard, its application ecosystem can build apps and tools that are more valuable because they are more interoperable.
Even without the semantic modeling tools, Snowflake needs to leverage the simplicity through integration of the tools built on its engine and metadata. For all the non-customer data Salesforce accounts have, Snowflake could continue to be the best platform. Salesforce can federate queries out to Snowflake when it wants to integrate that data. One example is the power of Cortex and all the gen AI functionality for working with unstructured data.
But the biggest issue is that customer analytics in the Salesforce platform can be integrated with outcomes in the Salesforce applications. The models can automatically learn from experience when it recommends an action that moves a lead successfully through a funnel, for example.
As much as Snowflake wants to be the simple, all-in-one iPhone platform for application developers, it’s still caught somewhere between being a great compute engine and a platform for intelligent operational applications that Salesforce Data Cloud has become. This is where we see gaps that Snowflake must fill over the next several months and years.
Snowflake is encroaching on Microsoft’s territory
Let’s now take a look at another approach which is highlighted by Microsoft Corp.’s data platform below.
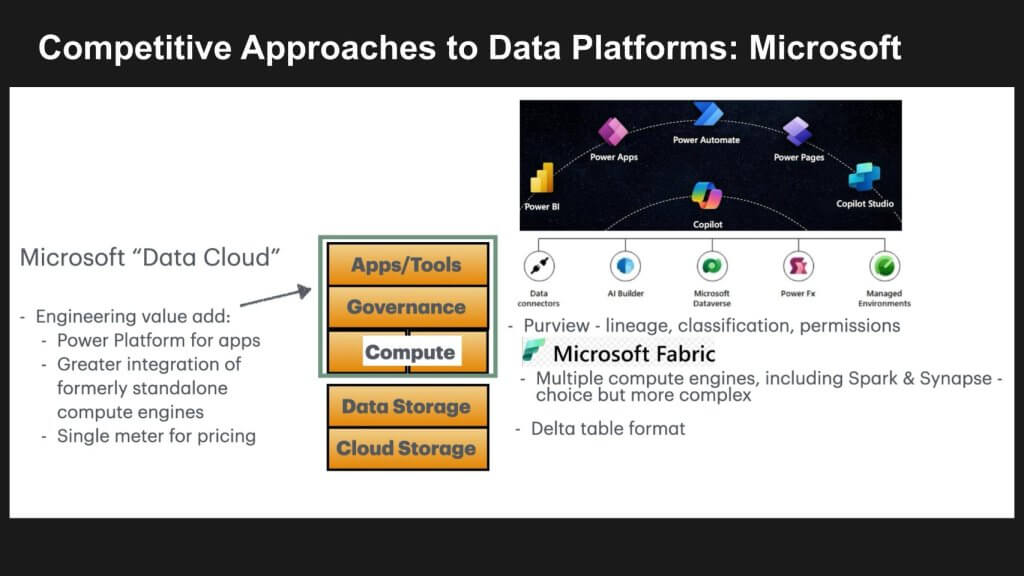
Microsoft doesn’t use the term data cloud, so we put that in quotes for consistency of comparison. But Microsoft has something that no other hyperscaler or data platform quite has, which is a rich no-code/low-code tool chain built up over a decade. Corporate developers can use it across Azure, Office and Dynamics. It has an abstraction layer which simplifies talking to all the Azure data services.
Microsoft has done the work to hide the differences in all its data services. This is in contrast to Amazon Web Services Inc., which has taken a right-tool-for-the-right-job approach with fine-grained primitives in each data service.
The Microsoft approach dramatically simplifies the equation for customers. At the compute layer with Fabric, Microsoft does have multiple engines, but our view is that they work together “well enough” that they can tell a coordinated story. Microsoft sales teams can lead with the simplicity and power of its tooling and drag a “good enough” Fabric along with it. Snowflake has the single unified engine and will lead with simplicity, but it doesn’t have the rich app dev tools yet.
Here’s the setup: Today, data is trapped in thousands of different apps with different semantics. Snowflake has a single unified engine and it can lead with simplicity; but it doesn’t have the maturity of app dev tools or the business logic coherency. In other words, it doesn’t have the capability to harmonize the meaning of data across the apps: “Billings” always means the same thing in each app. Salesforce, as we described above, doesn’t have that either, but it does have the advantage of owning the application logic. Microsoft has the rich app dev tool sets. No one firm has all three and the race is on.
Comparing the advantages of each approach
Snowflake is much richer, deeper and simpler than Microsoft Fabric. But Microsoft is trying to shift the purchase decision to a full-stack choice. It’s selling Power Platform as the way for corporate developers to build applications using Dynamics, Office and Azure as primitives. It’s selling the entire corporate product line as one virtual SKU. If customers want Snowflake, they can slot that in. But Microsoft is surrounding Snowflake and turning the iPhone into a component when they sell against it. That’s essentially what Salesforce has done, as well.
We see Snowflake’s encroachment on these new territories as a bold aspiration with many gaps, particularly with regard to preserving its core value prop and harmonizing data across its ecosystem to ensure interoperability. Over time its challenge is to fill those gaps with acquisitions, organic innovation and ecosystem partnerships. We see this as a critical next chapter in Snowflake’s history in order to preserve and growth its market value.
Customer spending profiles of Snowflake, Databricks and Salesforce
Let’s take a look at some survey research and some of the competitors in this race to the sixth data platform.
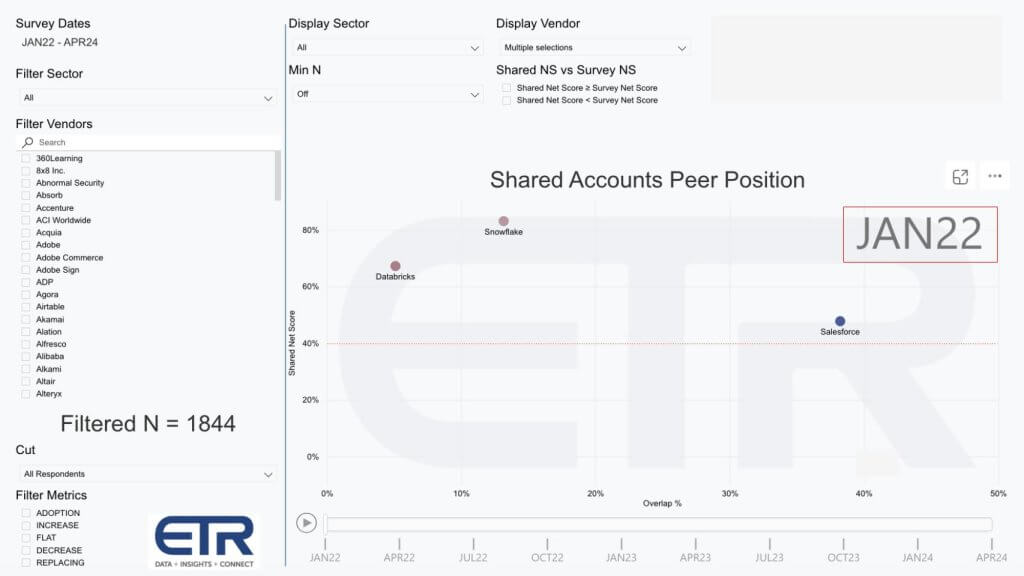
The data above goes back to the January 2022 Enterprise Technology Research survey of about 1,800 information technology decision makers. The vertical axis is Net Score, which is a measure of spending momentum. The horizontal axis represents the penetration or Overlap for each platform within that survey base. The red dotted line at 40% indicates a highly elevated spending velocity.
Note that Snowflake at the time was hitting record levels of Net Score at over 80%, meaning more than 80% of its customers were spending more on Snowflake after you net out those spending less or churning. Databricks was also very strong at the time with a Net Score in the mid-60s and Salesforce was also strong and above the 40% line… made even more impressive by its large penetration into the survey on the horizontal axis.
Fast-forward to April 2024
Snowflake accelerates, Databricks maintains momentum and expands its market presence
As we came out of the isolation economy, things were hot but they cooled off as the Fed tightened. And we saw IT spending growth rates slow and this hit many of the leading bellwether companies. But perhaps more interesting was the progression we saw in the relative positions of Snowflake and Databricks.
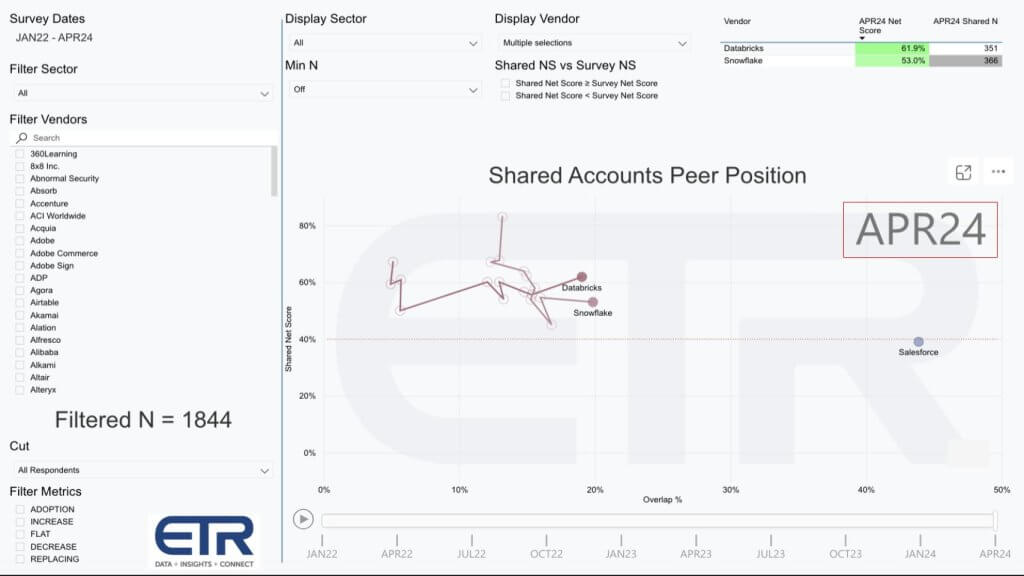
Look what happens above when we fast-forward 27 months to the April 2024 survey– follow the squiggly lines. While both Snowflake and Databricks are well above the 40% mark, Databricks’ market presence, when measured as a percent of customers adopting the platform, moves to the right – just behind Snowflake on the X axis. And Databricks’ spending velocity on the vertical axis is nearly 10 percentage points higher than that of Snowflake – 62% versus 53%.
Overlap of Databricks, Salesforce and Microsoft in Snowflake accounts
Given that we see Snowflake’s TAM expansion aspirations going headlong into not only Databricks accounts but also Salesforce and Microsoft accounts, we thought it would be interesting to look at the overlap of these players within Snowflake accounts. The chart below shows the same dimensions – Net Score or spending momentum on the Y axis and account Overlap on the X axis. But what we’ve done here is isolate on 425 Snowflake accounts.
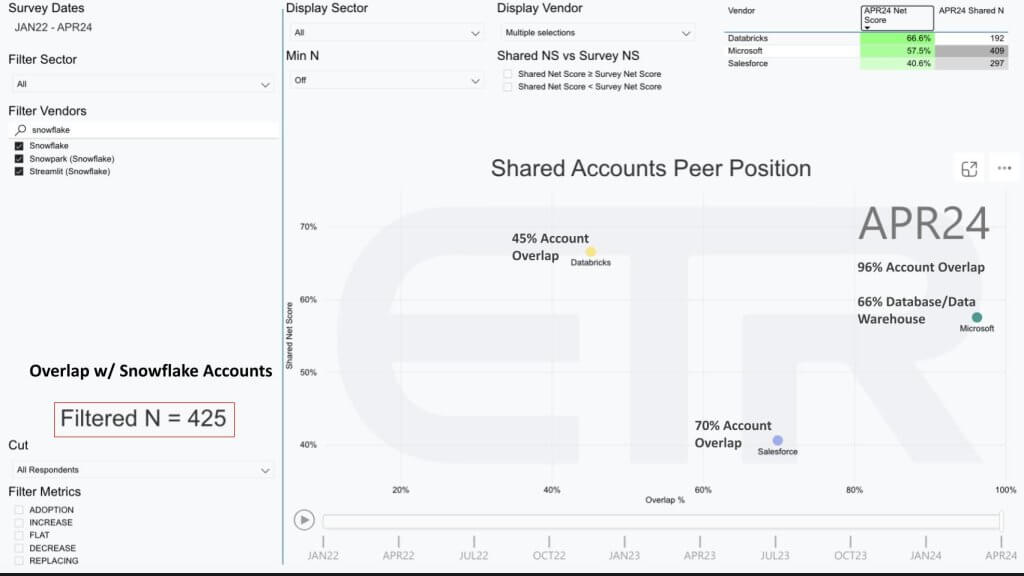
What the data tells us is that 45% of the 425 Snowflake accounts also have Databricks. Seventy percent have Salesforce and 96% are also Microsoft accounts. And 66% of those 425 Snowflake accounts also have Microsoft database or data warehouse products installed. Now, granted, this includes Microsoft operational databases, we don’t have the granularity to get to analytic databases only.
The point is that these competitors with the advantages we outlined have a major presence inside Snowflake accounts. So it presents both a go-to-market challenge and a technology innovation mandate for Snowflake.
Expectations for Snowflake Data Cloud Summit 2024
Let’s wrap up with what we want to see or will be watching at Snowflake Summit.
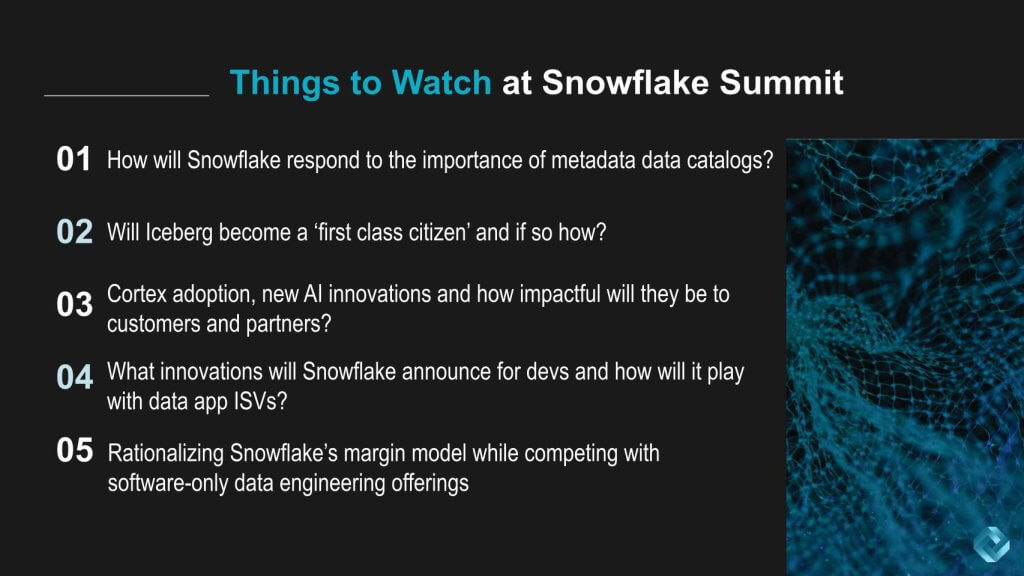
We’ll be watching how Snowflake and its customers are responding to the importance of metadata data catalogs. We firmly believe that the moat is shifting and it’s on Snowflake in our view not simply to respond, but to leapfrog and create new value vectors that it can protect and allow it to grow its valuation over time. That involves AI innovation, becoming the place to build trusted data apps.
Open source is a force. So will Iceberg become a “first-class citizen” and, if so, how? And what will that mean to Snowflake’s moat?
Cortex is Snowflake’s AI and ML platform. We’re interested in speaking to customers to understand their interest in adopting. But beyond Cortex, what new AI innovations will the company introduce? How will it leverage recent acquisitions and how impactful will these innovations be to customers and partners?
What innovations will Snowflake announce for developers and how will it play with data app independent software vendors? Specifically, how much enthusiasm is there around Snowpark and Snowpark Container Services? Remember, this is the first year that Snowflake is combining its developer event with its big customer event and we think that is a smart move and necessary to advance its value proposition.
And we really want to understand how Snowflake and its customers are rationalizing Snowflake’s margin model while competing with software-only data engineering offerings. Data engineering involves taking the raw data and turning it into intermediate data products, then outputs that business users can consume in the form of dashboards and increasingly AI models. And if customers are using tools outside Snowflake, it constricts their ability to validate the lineage.
The shift in data governance from being DBMS-centric to catalog-centric, exemplified by Databricks last year, aims to harmonize data across operational applications, ensuring consistent definitions — “customer” or “bookings.” Salesforce’s success in this area highlights the potential for Snowflake to advance by integrating gen AI across various user personas.
However, Snowflake faces challenges with its margin model and it needs to educate customers that data engineering workloads, which on the surface may appear costly because of marked-up infrastructure costs, actually are more efficient if performed inside Snowflake. Demonstrating superior total cost of ownership and trusted data governance remains a critical task for Snowflake.
Key points
- Shift to catalog-centric governance: Moving data governance from DBMS-centric to catalog-centric aligns with the trend of harmonizing data across operational applications.
- Salesforce example: Salesforce’s innovation in harmonizing data simplifies application building, a direction Snowflake should consider.
- Gen AI integration: Snowflake’s goal to integrate gen AI across all user personas must include a rich metadata catalog.
- Margin model sensitivity: Snowflake’s business model, which includes cloud infrastructure costs, makes data engineering workloads appear less price-competitive. Snowflake must combat this notion with clear proof points.
- Total cost of ownership: Snowflake needs to demonstrate that its total cost of ownership is superior, offering value in terms of trusted data and governance.
- Competitive landscape: Snowflake and Databricks’ events highlight their ongoing competition, with each company trying to position itself favorably. Salesforce and Microsoft are now vying for data platform dominance and the landscape is becoming more crowded due to the massive TAM.
Bottom line
These are all critical points and we will focus on the importance of transitioning to a catalog-centric approach to harmonize data definitions across applications. Snowflake’s enhanced gen AI offerings are critical to ensure great experiences for all users. The challenge lies in balancing the business model to make data engineering workloads cost-effective while proving superior total cost of ownership and governance. The competition between Snowflake and Databricks remains intense, new entrants with significant resources are coming into the mix and Snowflake must leverage its strengths to stay ahead.
What are you seeing in the market? How are you approaching your data engineering workloads? Snowflake wants to bring AI to your data… does that resonate? Do you see Snowflake as: 1) a data cloud; 2) an AI company; 3) a data application platform; or 4) all of the above.
Let us know what you think.
Your vote of support is important to us and it helps us keep the content FREE.
One click below supports our mission to provide free, deep, and relevant content.
Join our community on YouTube
Join the community that includes more than 15,000 #CubeAlumni experts, including Amazon.com CEO Andy Jassy, Dell Technologies founder and CEO Michael Dell, Intel CEO Pat Gelsinger, and many more luminaries and experts.
THANK YOU
Source link
lol