arXiv:2408.06356v1 Announce Type: new
Abstract: We introduce a unique semantic segmentation dataset of 6,096 high-resolution aerial images capturing indigenous and invasive grass species in Bega Valley, New South Wales, Australia, designed to address the underrepresented domain of ecological data in the computer vision community. This dataset presents a challenging task due to the overlap and distribution of grass species, which is critical for advancing models in ecological and agronomical applications. Our study features a homotopy-based multi-objective fine-tuning approach that balances segmentation accuracy and contextual consistency, applicable to various models. By integrating DiceCELoss for pixel-wise classification and a smoothness loss for spatial coherence, this method evolves during training to enhance robustness against noisy data. Performance baselines are established through a case study on the Segment Anything Model (SAM), demonstrating its effectiveness. Our annotation methodology, emphasizing pen size, zoom control, and memory management, ensures high-quality dataset creation. The dataset and code will be made publicly available, aiming to drive research in computer vision, machine learning, and ecological studies, advancing environmental monitoring and sustainable development.
Source link
lol
Enhancing Ecological Monitoring with Multi-Objective Optimization: A Novel Dataset and Methodology for Segmentation Algorithms
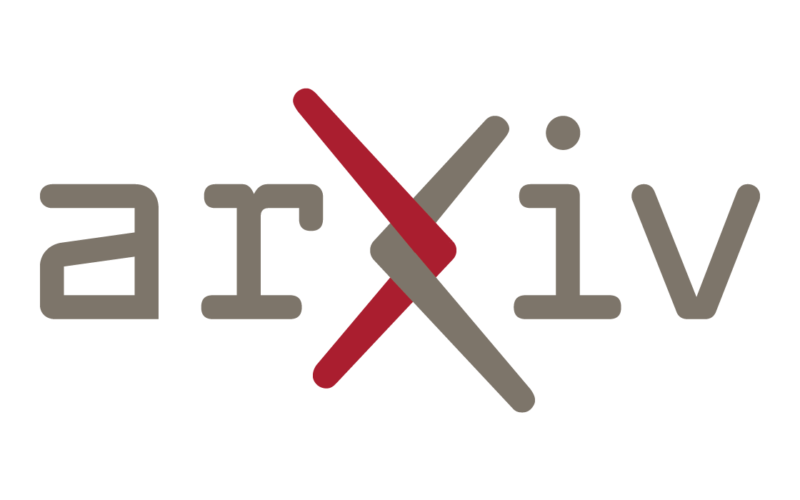