arXiv:2408.05440v1 Announce Type: new
Abstract: Implicit degradation modeling-based blind super-resolution (SR) has attracted more increasing attention in the community due to its excellent generalization to complex degradation scenarios and wide application range. How to extract more discriminative degradation representations and fully adapt them to specific image features is the key to this task. In this paper, we propose a new Content-decoupled Contrastive Learning-based blind image super-resolution (CdCL) framework following the typical blind SR pipeline. This framework introduces negative-free contrastive learning technique for the first time to model the implicit degradation representation, in which a new cyclic shift sampling strategy is designed to ensure decoupling between content features and degradation features from the data perspective, thereby improving the purity and discriminability of the learned implicit degradation space. In addition, to improve the efficiency and effectiveness of implicit degradation-based blind super-resolving, we design a detail-aware implicit degradation adaption module with lower complexity, which adapts degradation information to the specific LR image from both channel and spatial perspectives. Extensive experiments on synthetic and real data prove that the proposed CdCL comprehensively improves the quantitative and qualitative results of contrastive learning-based implicit blind SR paradigm, and achieves SOTA PSNR in this field. Even if the number of parameters is halved, our method still achieves very competitive results.
Source link
lol
Content-decoupled Contrastive Learning-based Implicit Degradation Modeling for Blind Image Super-Resolution
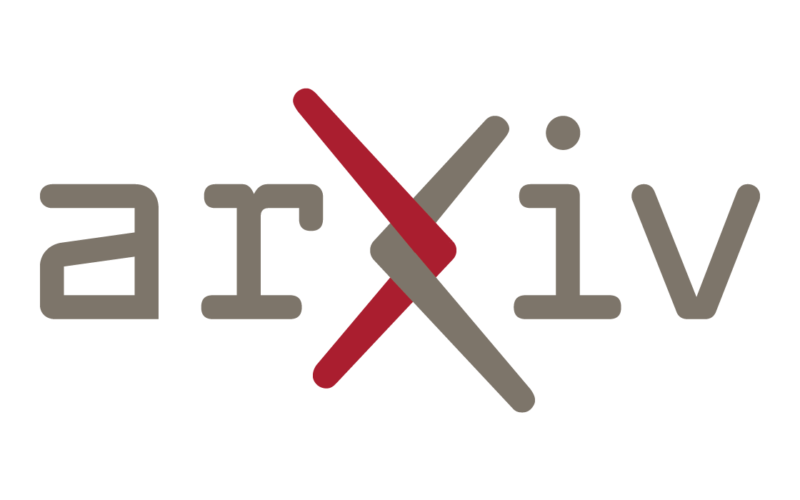