arXiv:2408.04823v1 Announce Type: new
Abstract: Infrared small target sequences exhibit strong similarities between frames and contain rich contextual information, which motivates us to achieve sequential infrared small target segmentation with minimal data. Inspired by the success of large segmentation models led by Segment Anything Model (SAM) across various downstream tasks, we propose a one-shot and training-free method that perfectly adapts SAM’s zero-shot generalization capabilities to sequential infrared small target segmentation. Given one annotated frame as a reference, our method can accurately segment small targets in other frames of the sequence. Specifically, we first obtain a confidence map through local feature matching between reference image and test image. Then, the highest point in the confidence map is as a prompt, and we design the Point Prompt-Centric Focusing (PPCF) module to address the over-segmentation of small targets with blurry boundaries. Subsequently, to prevent miss and false detections, we introduce the Triple-Level Ensemble (TLE) module that ensembles the masks obtained at different levels from the first two steps to produce the final mask. Experiments demonstrate that our method requires only one shot to achieve comparable performance to state-of-the-art methods based on traditional many-shot supervision and even superior performance in a few-shot setting. Moreover, ablation studies confirm the robustness of our approach to variations in one-shot samples, changes in scenes, and the presence of multiple targets.
Source link
lol
One Shot is Enough for Sequential Infrared Small Target Segmentation
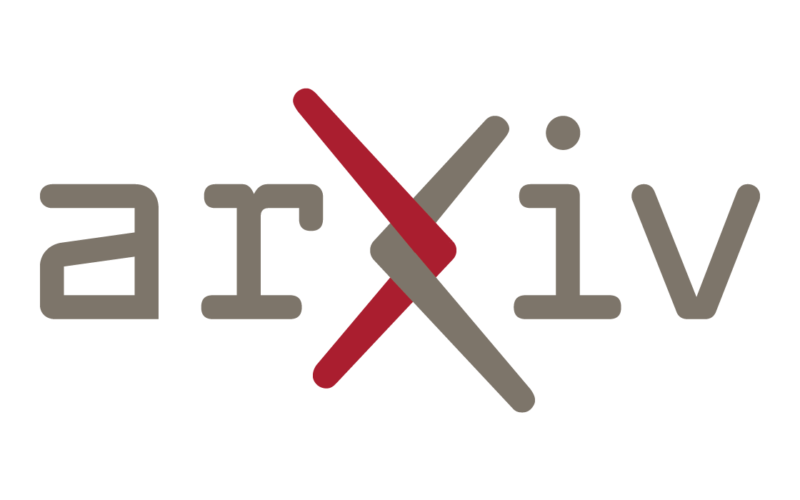