arXiv:2408.04251v1 Announce Type: new
Abstract: In a typical e-commerce setting, Content Ranking Optimization (CRO) mechanisms are employed to surface content on the search page to fulfill customers’ shopping missions. CRO commonly utilizes models such as contextual deep bandits model to independently rank content at different positions, e.g., one optimizer dedicated to organic search results and another to sponsored results. However, this regional optimization approach does not necessarily translate to whole page optimization, e.g., maximizing revenue at the top of the page may inadvertently diminish the revenue of lower positions. In this paper, we propose a reinforcement learning based method for whole page ranking to jointly optimize across all positions by: 1) shifting from position level optimization to whole page level optimization to achieve an overall optimized ranking; 2) applying reinforcement learning to optimize for the cumulative rewards instead of the instant reward. We formulate page level CRO as a cooperative Multi-agent Markov Decision Process , and address it with the novel Multi-Agent Deep Deterministic Policy Gradient (MADDPG) model. MADDPG supports a flexible and scalable joint optimization framework by adopting a “centralized training and decentralized execution” approach. Extensive experiments demonstrate that MADDPG scales to a 2.5 billion action space in the public Mujoco environment, and outperforms the deep bandits modeling by 25.7% on the offline CRO data set from a leading e-commerce company. We foresee that this novel multi-agent optimization is applicable to similar joint optimization problems in the field of information retrieval.
Source link
lol
Cooperative Multi-Agent Deep Reinforcement Learning in Content Ranking Optimization
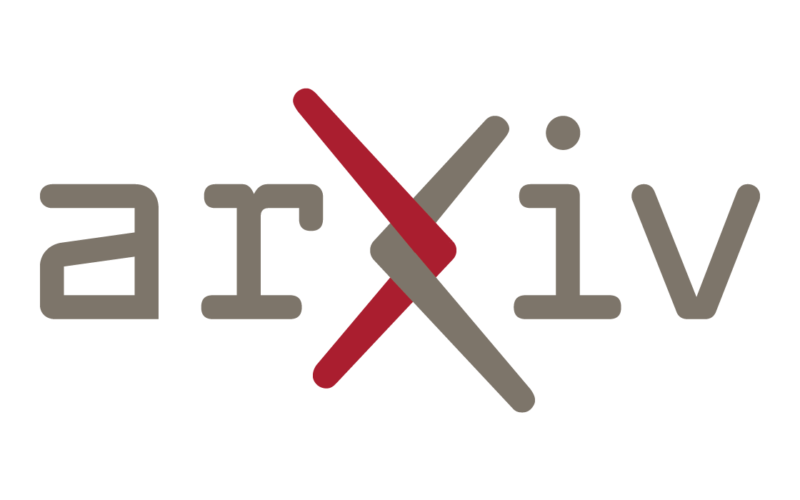