arXiv:2408.04206v1 Announce Type: new
Abstract: Sparse estimation for Gaussian graphical models is a crucial technique for making the relationships among numerous observed variables more interpretable and quantifiable. Various methods have been proposed, including graphical lasso, which utilizes the $ell_1$ norm as a regularization term, as well as methods employing non-convex regularization terms. However, most of these methods approximate the $ell_0$ norm with convex functions. To estimate more accurate solutions, it is desirable to treat the $ell_0$ norm directly as a regularization term. In this study, we formulate the sparse estimation problem for Gaussian graphical models using the $ell_0$ norm and propose a method to solve this problem using the Difference of Convex functions Algorithm (DCA). Specifically, we convert the $ell_0$ norm constraint into an equivalent largest-$K$ norm constraint, reformulate the constrained problem into a penalized form, and solve it using the DC algorithm (DCA). Furthermore, we designed an algorithm that efficiently computes using graphical lasso. Experimental results with synthetic data show that our method yields results that are equivalent to or better than existing methods. Comparisons of model learning through cross-validation confirm that our method is particularly advantageous in selecting true edges.
Source link
lol
DC Algorithm for Estimation of Sparse Gaussian Graphical Models
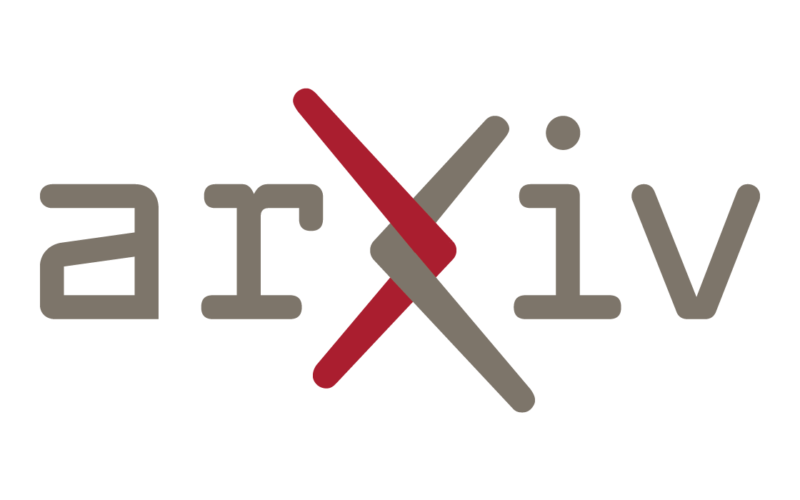