arXiv:2408.04211v1 Announce Type: new
Abstract: The importance of recommender systems is growing rapidly due to the exponential increase in the volume of content generated daily. This surge in content presents unique challenges for designing effective recommender systems. Key among these challenges is the need to effectively leverage the vast amounts of natural language data and images that represent user preferences. This paper presents a novel approach to enhancing recommender systems by leveraging Large Language Models (LLMs) and deep learning techniques. The proposed framework aims to improve the accuracy and relevance of recommendations by incorporating multi-modal information processing and by the use of unified latent space representation. The study explores the potential of LLMs to better understand and utilize natural language data in recommendation contexts, addressing the limitations of previous methods. The framework efficiently extracts and integrates text and image information through LLMs, unifying diverse modalities in a latent space to simplify the learning process for the ranking model. Experimental results demonstrate the enhanced discriminative power of the model when utilizing multi-modal information. This research contributes to the evolving field of recommender systems by showcasing the potential of LLMs and multi-modal data integration to create more personalized and contextually relevant recommendations.
Source link
lol
MMREC: LLM Based Multi-Modal Recommender System
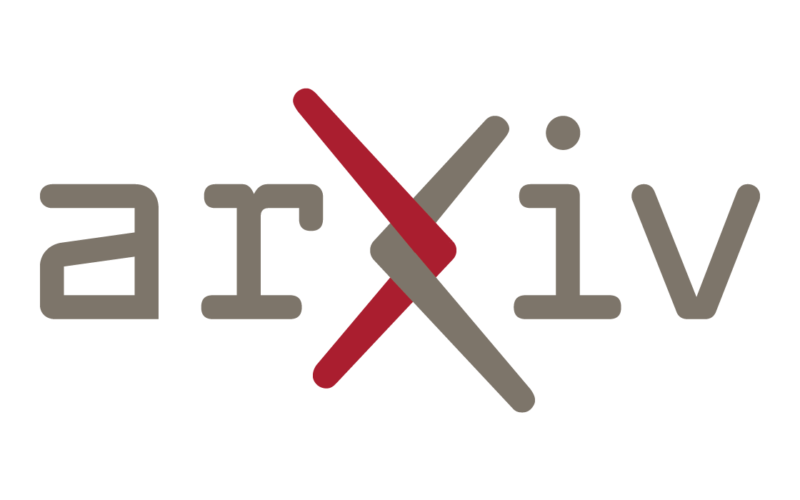