arXiv:2408.02692v1 Announce Type: new
Abstract: Effective flood hazard management requires evaluating and predicting flash flood susceptibility. Convolutional neural networks (CNNs) are commonly used for this task but face issues like gradient explosion and overfitting. This study explores the use of an attention mechanism, specifically the convolutional block attention module (CBAM), to enhance CNN models for flash flood susceptibility in the ungauged Rheraya watershed, a flood prone region. We used ResNet18, DenseNet121, and Xception as backbone architectures, integrating CBAM at different locations. Our dataset included 16 conditioning factors and 522 flash flood inventory points. Performance was evaluated using accuracy, precision, recall, F1-score, and the area under the curve (AUC) of the receiver operating characteristic (ROC). Results showed that CBAM significantly improved model performance, with DenseNet121 incorporating CBAM in each convolutional block achieving the best results (accuracy = 0.95, AUC = 0.98). Distance to river and drainage density were identified as key factors. These findings demonstrate the effectiveness of the attention mechanism in improving flash flood susceptibility modeling and offer valuable insights for disaster management.
Source link
lol
Attention is all you need for an improved CNN-based flash flood susceptibility modeling. The case of the ungauged Rheraya watershed, Morocco
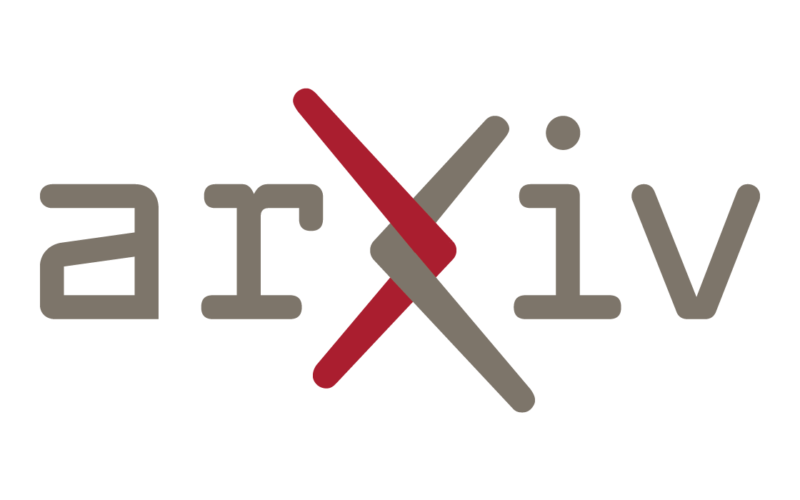