arXiv:2408.02683v1 Announce Type: new
Abstract: The early and accurate diagnosis of sepsis is critical for enhancing patient outcomes. This study aims to use heart rate variability (HRV) features to develop an effective predictive model for sepsis detection. Critical HRV features are identified through feature engineering methods, including statistical bootstrapping and the Boruta algorithm, after which XGBoost and Random Forest classifiers are trained with differential hyperparameter settings. In addition, ensemble models are constructed to pool the prediction probabilities of high-recall and high-precision classifiers and improve model performance. Finally, a neural network model is trained on the HRV features, achieving an F1 score of 0.805, a precision of 0.851, and a recall of 0.763. The best-performing machine learning model is compared to this neural network through an interpretability analysis, where Local Interpretable Model-agnostic Explanations are implemented to determine decision-making criterion based on numerical ranges and thresholds for specific features. This study not only highlights the efficacy of HRV in automated sepsis diagnosis but also increases the transparency of black box outputs, maximizing clinical applicability.
Source link
lol
Improving Machine Learning Based Sepsis Diagnosis Using Heart Rate Variability
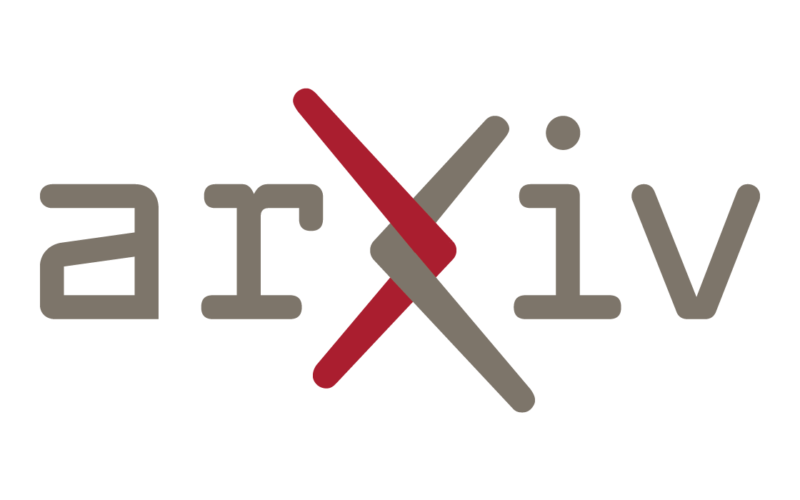