arXiv:2408.02676v1 Announce Type: new
Abstract: Recent work has uncovered alarming disparities in the performance of machine learning models in healthcare. In this study, we explore whether such disparities are present in the UK Biobank fundus retinal images by training and evaluating a disease classification model on these images. We assess possible disparities across various population groups and find substantial differences despite strong overall performance of the model. In particular, we discover unfair performance for certain assessment centres, which is surprising given the rigorous data standardisation protocol. We compare how these differences emerge and apply a range of existing bias mitigation methods to each one. A key insight is that each disparity has unique properties and responds differently to the mitigation methods. We also find that these methods are largely unable to enhance fairness, highlighting the need for better bias mitigation methods tailored to the specific type of bias.
Source link
lol
On Biases in a UK Biobank-based Retinal Image Classification Model
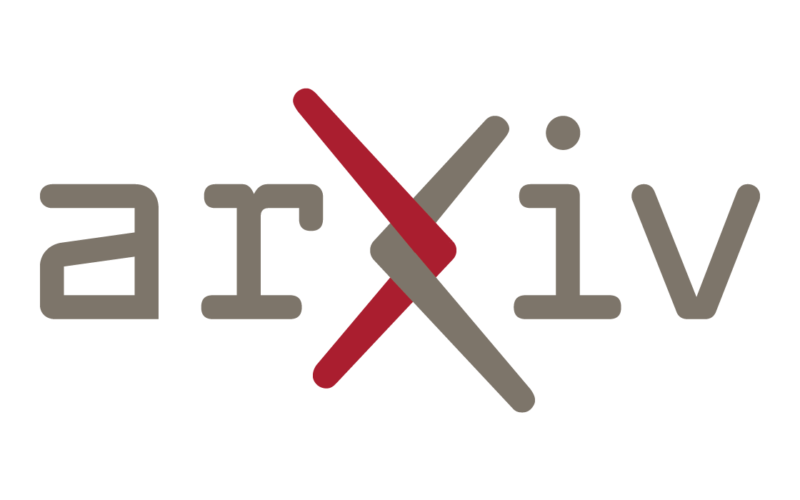