arXiv:2408.01612v1 Announce Type: new
Abstract: Background: Sepsis is a severe condition responsible for many deaths worldwide. Accurate prediction of sepsis outcomes is crucial for timely and effective treatment. Although previous studies have used ML to forecast outcomes, they faced limitations in feature selection and model comprehensibility, resulting in less effective predictions. Thus, this research aims to develop an interpretable and accurate ML model to help clinical professionals predict in-hospital mortality.
Methods: We analyzed ICU patient records from the MIMIC-III database based on specific criteria and extracted relevant data. Our feature selection process included a literature review, clinical input refinement, and using Random Forest to select the top 35 features. We performed data preprocessing, including cleaning, imputation, standardization, and applied SMOTE for oversampling to address imbalance, resulting in 4,683 patients, with admission counts of 17,429. We compared the performance of Random Forest, Gradient Boosting, Logistic Regression, SVM, and KNN models.
Results: The Random Forest model was the most effective in predicting sepsis-related in-hospital mortality. It outperformed other models, achieving an accuracy of 0.90 and an AUROC of 0.97, significantly better than the existing literature. Our meticulous feature selection contributed to the model’s precision and identified critical determinants of sepsis mortality. These results underscore the pivotal role of data-driven ML in healthcare, especially for predicting in-hospital mortality due to sepsis.
Conclusion: This study represents a significant advancement in predicting in-hospital sepsis mortality, highlighting the potential of ML in healthcare. The implications are profound, offering a data-driven approach that enhances decision-making in patient care and reduces in-hospital mortality.
Source link
lol
Data-Driven Machine Learning Approaches for Predicting In-Hospital Sepsis Mortality
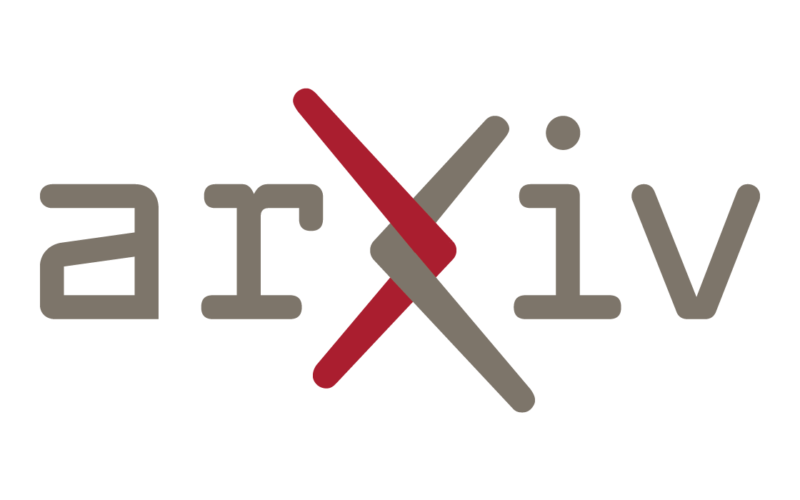