arXiv:2408.01517v1 Announce Type: new
Abstract: We prove that the usual gradient flow in parameter space that underlies many training algorithms for neural networks in deep learning can be continuously deformed into an adapted gradient flow which yields (constrained) Euclidean gradient flow in output space. Moreover, if the Jacobian of the outputs with respect to the parameters is full rank (for fixed training data), then the time variable can be reparametrized so that the resulting flow is simply linear interpolation, and a global minimum can be achieved.
Source link
lol
Gradient flow in parameter space is equivalent to linear interpolation in output space
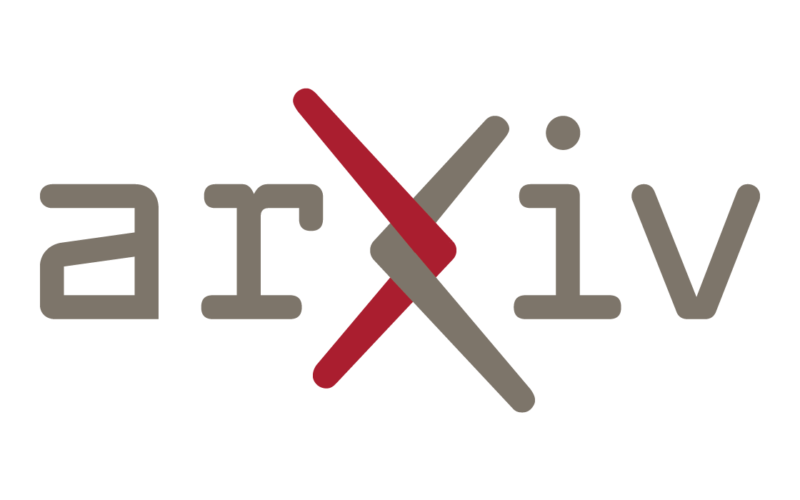