arXiv:2408.01427v1 Announce Type: new
Abstract: Humans exhibit remarkable proficiency in visual classification tasks, accurately recognizing and classifying new images with minimal examples. This ability is attributed to their capacity to focus on details and identify common features between previously seen and new images. In contrast, existing few-shot image classification methods often emphasize either global features or local features, with few studies considering the integration of both. To address this limitation, we propose a novel approach based on the Siamese Transformer Network (STN). Our method employs two parallel branch networks utilizing the pre-trained Vision Transformer (ViT) architecture to extract global and local features, respectively. Specifically, we implement the ViT-Small network architecture and initialize the branch networks with pre-trained model parameters obtained through self-supervised learning. We apply the Euclidean distance measure to the global features and the Kullback-Leibler (KL) divergence measure to the local features. To integrate the two metrics, we first employ L2 normalization and then weight the normalized results to obtain the final similarity score. This strategy leverages the advantages of both global and local features while ensuring their complementary benefits. During the training phase, we adopt a meta-learning approach to fine-tune the entire network. Our strategy effectively harnesses the potential of global and local features in few-shot image classification, circumventing the need for complex feature adaptation modules and enhancing the model’s generalization ability. Extensive experiments demonstrate that our framework is simple yet effective, achieving superior performance compared to state-of-the-art baselines on four popular few-shot classification benchmarks in both 5-shot and 1-shot scenarios.
Source link
lol
Siamese Transformer Networks for Few-shot Image Classification
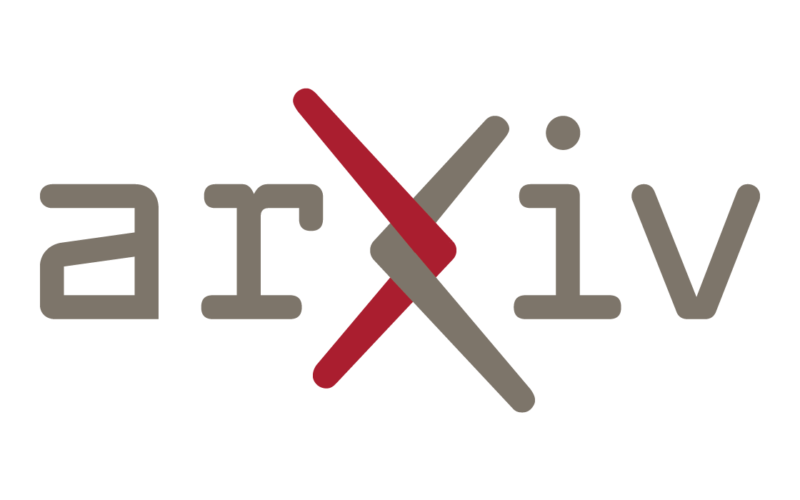