arXiv:2407.19183v1 Announce Type: new
Abstract: Graph data in real-world scenarios undergo rapid and frequent changes, making it challenging for existing graph models to effectively handle the continuous influx of new data and accommodate data withdrawal requests. The approach to frequently retraining graph models is resource intensive and impractical. To address this pressing challenge, this paper introduces a new concept of graph memory learning. Its core idea is to enable a graph model to selectively remember new knowledge but forget old knowledge. Building on this approach, the paper presents a novel graph memory learning framework – Brain-inspired Graph Memory Learning (BGML), inspired by brain network dynamics and function-structure coupling strategies. BGML incorporates a multi-granular hierarchical progressive learning mechanism rooted in feature graph grain learning to mitigate potential conflict between memorization and forgetting in graph memory learning. This mechanism allows for a comprehensive and multi-level perception of local details within evolving graphs. In addition, to tackle the issue of unreliable structures in newly added incremental information, the paper introduces an information self-assessment ownership mechanism. This mechanism not only facilitates the propagation of incremental information within the model but also effectively preserves the integrity of past experiences. We design five types of graph memory learning tasks: regular, memory, unlearning, data-incremental, and class-incremental to evaluate BGML. Its excellent performance is confirmed through extensive experiments on multiple real-world node classification datasets.
Source link
lol
Graph Memory Learning: Imitating Lifelong Remembering and Forgetting of Brain Networks
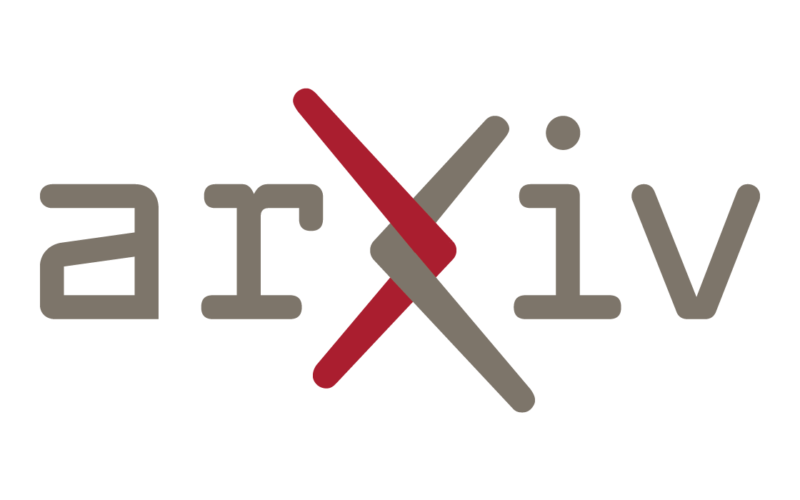