arXiv:2407.19119v1 Announce Type: new
Abstract: Over the last few years, federated learning (FL) has emerged as a prominent method in machine learning, emphasizing privacy preservation by allowing multiple clients to collaboratively build a model while keeping their training data private. Despite this focus on privacy, FL models are susceptible to various attacks, including membership inference attacks (MIAs), posing a serious threat to data confidentiality. In a recent study, Rezaei textit{et al.} revealed the existence of an accuracy-privacy trade-off in deep ensembles and proposed a few fusion strategies to overcome it. In this paper, we aim to explore the relationship between deep ensembles and FL. Specifically, we investigate whether confidence-based metrics derived from deep ensembles apply to FL and whether there is a trade-off between accuracy and privacy in FL with respect to MIA. Empirical investigations illustrate a lack of a non-monotonic correlation between the number of clients and the accuracy-privacy trade-off. By experimenting with different numbers of federated clients, datasets, and confidence-metric-based fusion strategies, we identify and analytically justify the clear existence of the accuracy-privacy trade-off.
Source link
lol
Accuracy-Privacy Trade-off in the Mitigation of Membership Inference Attack in Federated Learning
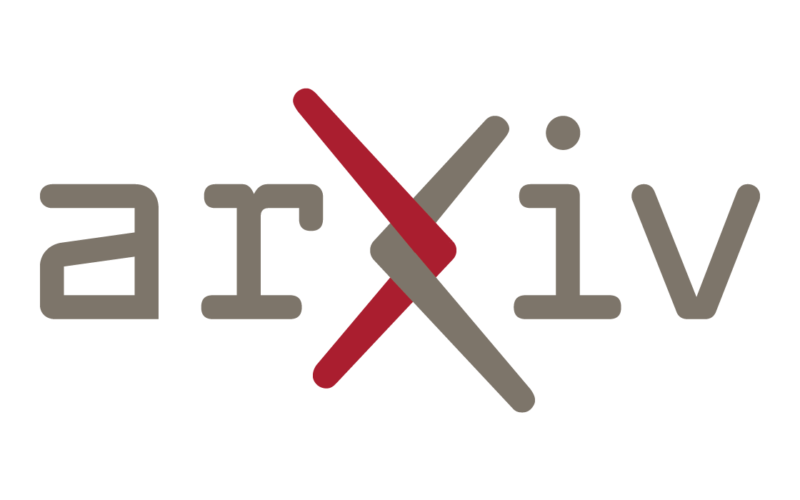