arXiv:2407.18941v1 Announce Type: new
Abstract: Large repositories of image-caption pairs are essential for the development of vision-language models. However, these datasets are often extracted from noisy data scraped from the web, and contain many mislabeled examples. In order to improve the reliability of downstream models, it is important to identify and filter images with incorrect captions. However, beyond filtering based on image-caption embedding similarity, no prior works have proposed other methods to filter noisy multimodal data, or concretely assessed the impact of noisy captioning data on downstream training. In this work, we propose LEMoN, a method to automatically identify label errors in multimodal datasets. Our method leverages the multimodal neighborhood of image-caption pairs in the latent space of contrastively pretrained multimodal models. We find that our method outperforms the baselines in label error identification, and that training on datasets filtered using our method improves downstream classification and captioning performance.
Source link
lol
LEMoN: Label Error Detection using Multimodal Neighbors
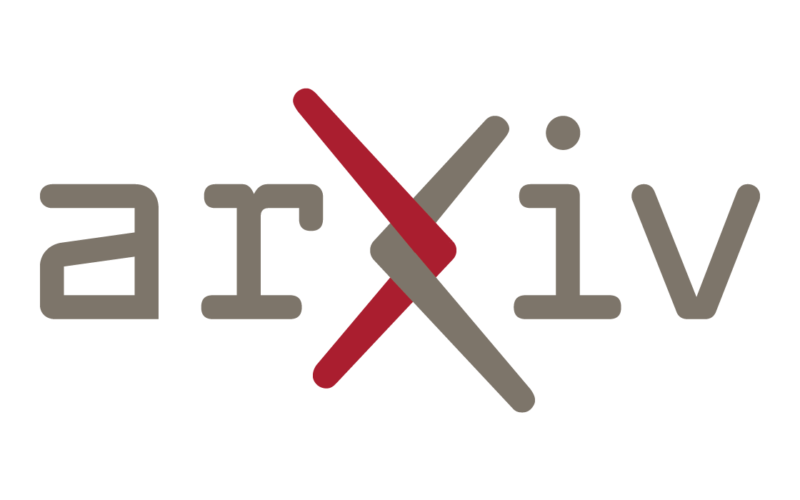