arXiv:2407.18324v1 Announce Type: new
Abstract: Stock volatility prediction is an important task in the financial industry. Recent advancements in multimodal methodologies, which integrate both textual and auditory data, have demonstrated significant improvements in this domain, such as earnings calls (Earnings calls are public available and often involve the management team of a public company and interested parties to discuss the company’s earnings). However, these multimodal methods have faced two drawbacks. First, they often fail to yield reliable models and overfit the data due to their absorption of stochastic information from the stock market. Moreover, using multimodal models to predict stock volatility suffers from gender bias and lacks an efficient way to eliminate such bias. To address these aforementioned problems, we use adversarial training to generate perturbations that simulate the inherent stochasticity and bias, by creating areas resistant to random information around the input space to improve model robustness and fairness. Our comprehensive experiments on two real-world financial audio datasets reveal that this method exceeds the performance of current state-of-the-art solution. This confirms the value of adversarial training in reducing stochasticity and bias for stock volatility prediction tasks.
Source link
lol
AMA-LSTM: Pioneering Robust and Fair Financial Audio Analysis for Stock Volatility Prediction
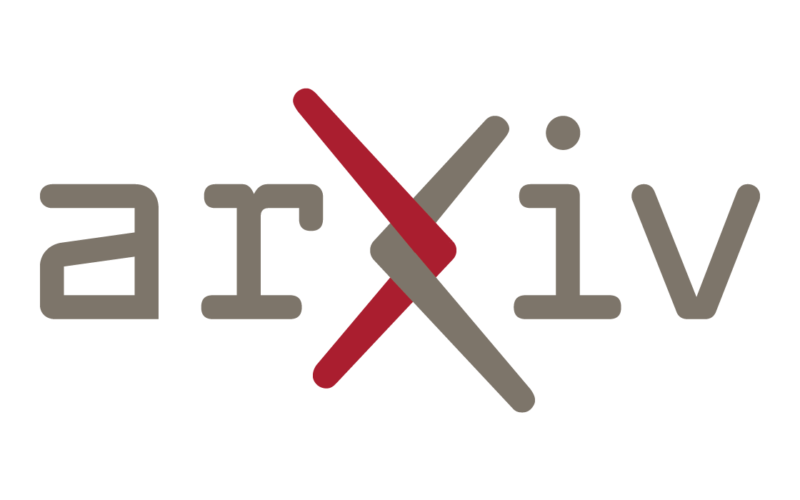