(Anders78/Shutterstock)
The advent of generative AI has supercharged the world’s appetite for data, especially high-quality data of known provenance. However, as large language models (LLMs) get bigger, experts are warning that we may be running out of data to train them.
One of the big shifts that occurred with transformer models, which were invented by Google in 2017, is the use of unsupervised learning. Instead of training an AI model in a supervised fashion atop smaller amounts of higher quality, human-curated data, the use of unsupervised training with transformer models opened AI up to the vast amounts of data of variable quality on the Web.
As pre-trained LLMs have gotten bigger and more capable over the years, they have required bigger and more elaborate training sets. For instance, when OpenAI released its original GPT-1 model in 2018, the model had about 115 million parameters and was trained on BookCorpus, which is a collection of about 7,000 unpublished books comprising about 4.5 GB of text.
GPT-2, which OpenAI launched in 2019, represented a direct 10x scale-up of GPT-1. The parameter count expanded to 1.5 billion and the training data expanded to about 40GB via the company’s use of WebText, a novel training set it created based on scraped links from Reddit users. WebText contained about 600 billion words and weighed in around 40GB.
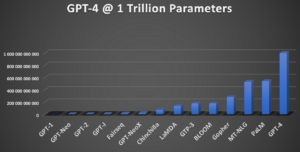
LLM growth by number of parameters (Image courtesy Corus Greyling, HumanFirst)
With GPT-3, OpenAI expanded its parameter count to 175 billion. The model, which debuted in 2020, was pre-trained on 570 GB of text culled from open sources, including BookCorpus (Book1 and Book2), Common Crawl, Wikipedia, and WebText2. All told, it amounted to about 499 billion tokens.
While official size and training set details are scant for GPT-4, which OpenAI debuted in 2023, estimates peg the size of the LLM at somewhere between 1 trillion and 1.8 trillion, which would make it five to 10 times bigger than GPT-3. The training set, meanwhile, has been reported to be 13 trillion tokens (roughly 10 trillion words).
As the AI models get bigger, the AI model makers have scoured the Web for new sources of data to train them. However, that is getting harder, as the creators and collectors of Web data have increasingly imposed restrictions on the use of data for training AI.
Dario Amodei, the CEO of Anthropic, recently estimated there’s a 10% chance that we could run out of enough data to continue scaling models.
“…[W]e could run out of data,” Amodei told Dwarkesh Patel in a recent interview. “For various reasons, I think that’s not going to happen but if you look at it very naively we’re not that far from running out of data.”
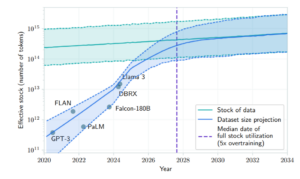
We will soon use up all novel human text data for LLM training, researchers say (Will we run out of data? Limits of LLM scaling based on human-generated data”)
This topic was also taken up in a recent paper titled “Will we run out of data? Limits of LLM scaling based on human-generated data,” where researchers suggest that the current pace of LLM development on human-based data is not sustainable.
At current rates of scaling, an LLM that is trained on all available human text data will be created between 2026 and 2032, they wrote. In other words, we could run out of fresh data that no LLM has seen in less than two years.
“However, after accounting for steady improvements in data efficiency and the promise of techniques like transfer learning and synthetic data generation, it is likely that we will be
able to overcome this bottleneck in the availability of public
human text data,” the researchers write.
In a new paper from the Data Provenance Initiative titled “Consent in Crisis: The Rapid Decline of the AI Data Commons” (pdf), researchers affiliated with the Massachusetts Institute of Technology analyzed 14,000 websites to determine to what extent website operators are making their data “crawlable” by automated data harvesters, such as those used by Common Crawl, the largest publicly available crawl of the Internet.
Their conclusion: Much of the data increasingly is off-limits to Web crawlers, either by policy or technological incompatibility. What’s more, the terms of use dictating how website operators’ allow their data to be used increasingly don’t mesh with what websites actually allow through their robot.txt files, which contain rules that block access to content.
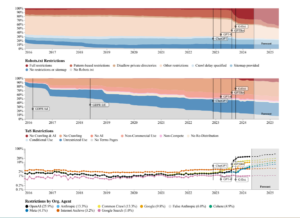
Website operators are putting restrictions on data harvesting (Courtesy “Consent in Crisis: The Rapid Decline of the AI Data Commons”)
“We observe a proliferation of AI-specific clauses to limit use, acute differences in restrictions on AI developers, as well as general inconsistencies between websites’ expressed intentions in their Terms of Service and their robots.txt,” the Data Provenance Initiative researchers wrote. “We diagnose these as symptoms of ineffective web protocols, not designed to cope with the widespread re-purposing of the internet for AI.”
Common Crawl has been recording the Internet since 2007, and today is composed of more than 250 billion Web pages. The repository is free and open for anyone to use, and grows by 3 billion to 5 billion new pages per month. Groups like C4, RefinedWeb, and Dolma, which were analyzed by the MIT researchers, offer cleaned up versions of the data in Common Crawl.
The Data Provenance Initiative researchers found that, since OpenAI’s ChatGPT exploded onto the scene in late 2022, many websites have imposed restrictions on crawling for the purpose of harvesting data. At current rates, nearly 50% of websites are projected to have full or partial restrictions by 2025, the researchers conclude. Similarly, restrictions have also been imposed on website terms of service (ToS), with the percentage of websites with no restrictions dropping from about 50% in 2023 to about 40% by 2025.
The Data Provenance Initiative researchers find that crawlers from OpenAI are restricted the most often, about 26% of the time, followed by crawlers from Anthropic and Common Crawl (about 13%), Google’s AI crawler (about 10%), Cohere (about 5%), and Meta (about 4%).
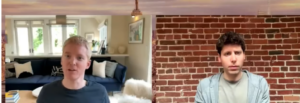
Patrick Collison interviews OpenAI CEO Sam Altman
The Internet was not created to provide data for training AI models, the researchers write. While larger websites are able to implement sophisticated consent controls that allow them to expose some data sets with full provenance while retricting others, many smaller websites operators don’t have the resources to implement such systems, which means they’re hiding all of their content behind paywalls, the researchers write. That prevents AI companies from getting to it, but it also prevents that data from being used for more legitimate uses, such as academic research, taking us further from the Internet’s open beginnings.
“If we don’t develop better mechanisms to give website owners control over how their data is used, we should expect to see further decreases in the open web,” the Data Provenance Initiative researchers write.
AI giants have recently started to look to other sources for data to train their models, including huge collections of videos posted to the Internet. For instance, a dataset called YouTube Subtitles, which is part of larger, open-source data set created by EleutherAI called the Pile, is being used by companies like Apple, Nvidia, and Anthropic to train AI models.
The move has angered some smaller content creators, who say they never agreed to have their copyrighted work used to train AI models and have not been compensated as such. What’s more, they have expressed concern that their content may be used to train generative models that create content that competes with their own content.
The AI companies are aware of the looming data dam, but they have potentials workarounds already in the works. OpenAI CEO Sam Altman acknowledged the situation in a recent interview with Irish entrepreneur Patrick Collison.
“As long as you can get over the synthetic data event horizon where the model is smart enough to create synthetic data, I think it will be alright,” Altman said. “We do need new techniques for sure. I don’t want to pretend otherwise in any way. But the naïve plan of scaling up a transformer with pre-trained tokens from the Internet–that will run out. But that’s not the plan.”
Related Items:
Are Tech Giants ‘Piling’ On Small Content Creators to Train Their AI?
Anger Builds Over Big Tech’s Big Data Abuses
curation, data provenance, GenAI, human data, LLM, provenance, synthetic data, text data, training data, training dataset, transformer model
Source link
lol