arXiv:2407.16772v1 Announce Type: new
Abstract: Fine-grained understanding of objects, attributes, and relationships between objects is crucial for visual-language models (VLMs). Existing benchmarks primarily focus on evaluating VLMs’ capability to distinguish between two very similar textit{captions} given an image. In this paper, we introduce a new, challenging benchmark termed textbf{Vis}ual textbf{Min}imal-Change Understanding (VisMin), which requires models to predict the correct image-caption match given two images and two captions. The image pair and caption pair contain minimal changes, i.e., only one aspect changes at a time from among the following: textit{object}, textit{attribute}, textit{count}, and textit{spatial relation}. These changes test the models’ understanding of objects, attributes (such as color, material, shape), counts, and spatial relationships between objects. We built an automatic framework using large language models and diffusion models, followed by a rigorous 4-step verification process by human annotators. Empirical experiments reveal that current VLMs exhibit notable deficiencies in understanding spatial relationships and counting abilities. We also generate a large-scale training dataset to finetune CLIP and Idefics2, showing significant improvements in fine-grained understanding across benchmarks and in CLIP’s general image-text alignment. We release all resources, including the benchmark, training data, and finetuned model checkpoints, at url{https://vismin.net/}.
Source link
lol
VisMin: Visual Minimal-Change Understanding
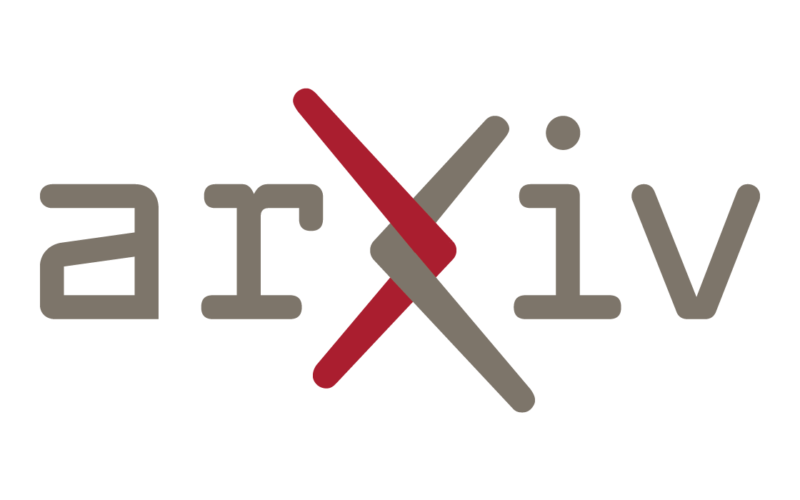