arXiv:2407.16125v1 Announce Type: new
Abstract: Recent studies on inverse problems have proposed posterior samplers that leverage the pre-trained diffusion models as powerful priors. These attempts have paved the way for using diffusion models in a wide range of inverse problems. However, the existing methods entail computationally demanding iterative sampling procedures and optimize a separate solution for each measurement, which leads to limited scalability and lack of generalization capability across unseen samples. To address these limitations, we propose a novel approach, Diffusion prior-based Amortized Variational Inference (DAVI) that solves inverse problems with a diffusion prior from an amortized variational inference perspective. Specifically, instead of separate measurement-wise optimization, our amortized inference learns a function that directly maps measurements to the implicit posterior distributions of corresponding clean data, enabling a single-step posterior sampling even for unseen measurements. Extensive experiments on image restoration tasks, e.g., Gaussian deblur, 4$times$ super-resolution, and box inpainting with two benchmark datasets, demonstrate our approach’s superior performance over strong baselines. Code is available at https://github.com/mlvlab/DAVI.
Source link
lol
Diffusion Prior-Based Amortized Variational Inference for Noisy Inverse Problems
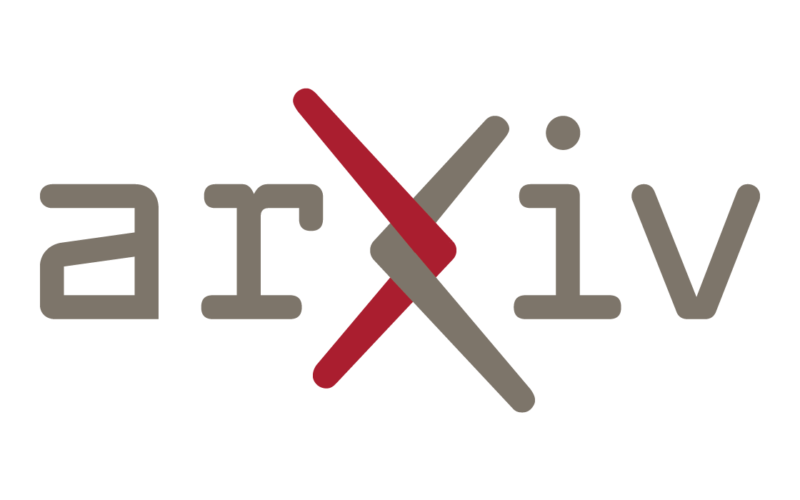