arXiv:2407.15869v1 Announce Type: new
Abstract: Short fixed-length inputs are the main bottleneck of deep learning methods in long time-series forecasting tasks. Prolonging input length causes overfitting, rapidly deteriorating accuracy. Our research indicates that the overfitting is a combination reaction of the multi-scale pattern coupling in time series and the fixed focusing scale of current models. First, we find that the patterns exhibited by a time series across various scales are reflective of its multi-periodic nature, where each scale corresponds to specific period length. Second, We find that the token size predominantly dictates model behavior, as it determines the scale at which the model focuses and the context size it can accommodate. Our idea is to decouple the multi-scale temporal patterns of time series and to model each pattern with its corresponding period length as token size. We introduced a novel series-decomposition module(MPSD), and a Multi-Token Pattern Recognition neural network(MTPR), enabling the model to handle textit{inputs up to $10times$ longer}. Sufficient context enhances performance(textit{38% maximum precision improvement}), and the decoupling approach offers textit{Low complexity($0.22times$ cost)} and textit{high interpretability}.
Source link
lol
Long Input Sequence Network for Long Time Series Forecasting
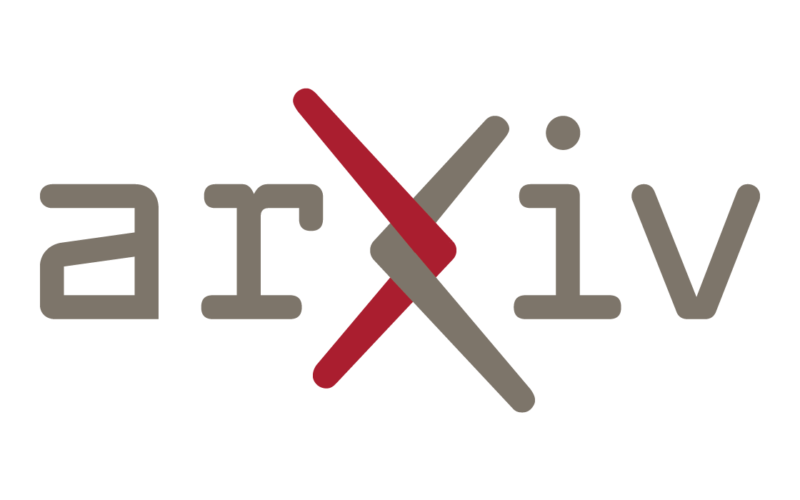