arXiv:2407.13808v1 Announce Type: new
Abstract: We propose a novel prompt tuning method called CoAPT(Context Attribute words in Prompt Tuning) for few/zero-shot image classification. The core motivation is that attributes are descriptive words with rich information about a given concept. Thus, we aim to enrich text queries of existing prompt tuning methods, improving alignment between text and image embeddings in CLIP embedding space. To do so, CoAPT integrates attribute words as additional prompts within learnable prompt tuning and can be easily incorporated into various existing prompt tuning methods. To facilitate the incorporation of attributes into text embeddings and the alignment with image embeddings, soft prompts are trained together with an additional meta-network that generates input-image-wise feature biases from the concatenated feature encodings of the image-text combined queries. Our experiments demonstrate that CoAPT leads to considerable improvements for existing baseline methods on several few/zero-shot image classification tasks, including base-to-novel generalization, cross-dataset transfer, and domain generalization. Our findings highlight the importance of combining hard and soft prompts and pave the way for future research on the interplay between text and image latent spaces in pre-trained models.
Source link
lol
CoAPT: Context Attribute words for Prompt Tuning
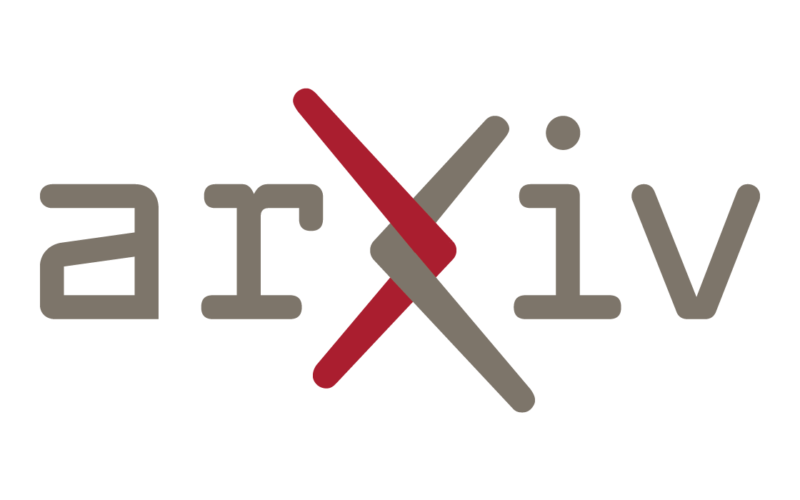