arXiv:2407.13806v1 Announce Type: new
Abstract: Current Transformer methods for Multivariate Time-Series Forecasting (MTSF) are all based on the conventional attention mechanism. They involve sequence embedding and performing a linear projection of Q, K, and V, and then computing attention within this latent space. We have never delved into the attention mechanism to explore whether such a mapping space is optimal for MTSF. To investigate this issue, this study first proposes Frequency Spectrum attention (FSatten), a novel attention mechanism based on the frequency domain space. It employs the Fourier transform for embedding and introduces Multi-head Spectrum Scaling (MSS) to replace the conventional linear mapping of Q and K. FSatten can accurately capture the periodic dependencies between sequences and outperform the conventional attention without changing mainstream architectures. We further design a more general method dubbed Scaled Orthogonal attention (SOatten). We propose an orthogonal embedding and a Head-Coupling Convolution (HCC) based on the neighboring similarity bias to guide the model in learning comprehensive dependency patterns. Experiments show that FSatten and SOatten surpass the SOTA which uses conventional attention, making it a good alternative as a basic attention mechanism for MTSF. The codes and log files will be released at: https://github.com/Joeland4/FSatten-SOatten.
Source link
lol
Revisiting Attention for Multivariate Time Series Forecasting
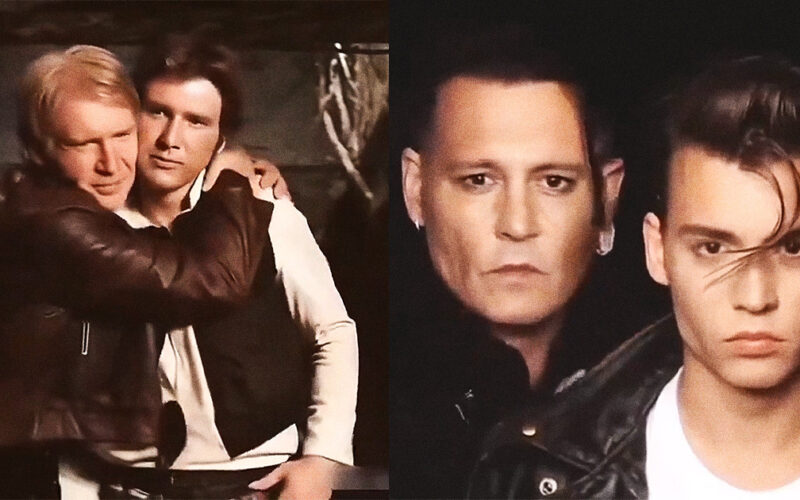