arXiv:2407.13781v1 Announce Type: new
Abstract: Recently, various encoder-only and encoder-decoder pre-trained models like BERT and T5 have been applied to automatic essay scoring (AES) as small language models. However, existing studies have primarily treated this task akin to a classification problem, focusing solely on outputting scores in the target text without offering interpretations for the generated scores. Departing from the approaches, we introduce Reasoning Distillation-Based Evaluation (RDBE), which integrates interpretability to elucidate the rationale behind model scores while enhancing performance through initial reasoning. This interpretive capability is acquired during training by leveraging generated reasoning from a large language model (LLM) to distill a small language model (SLM). Our experimental results demonstrate the efficacy of RDBE across all scoring rubrics considered in the dataset. RDBE outperforms both zero-shot LLM generation and generation from a baseline fine-tuned model, establishing itself as state-of-the-art in the corresponding dataset. This highlights its practical interpretative output and enhanced performance.
Source link
lol
RDBE: Reasoning Distillation-Based Evaluation Enhances Automatic Essay Scoring
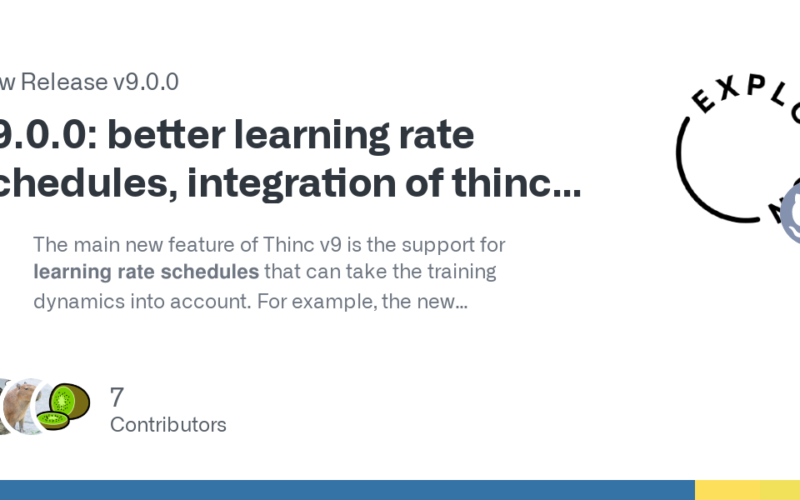