arXiv:2407.12018v1 Announce Type: new
Abstract: Despite the extensive communication benefits offered by social media platforms, numerous challenges must be addressed to ensure user safety. One of the most significant risks faced by users on these platforms is targeted hate speech. Social media platforms are widely utilised for generating datasets employed in training and evaluating machine learning algorithms for hate speech detection. However, existing public datasets exhibit numerous limitations, hindering the effective training of these algorithms and leading to inaccurate hate speech classification. This study provides a comprehensive empirical evaluation of several public datasets commonly used in automated hate speech classification. Through rigorous analysis, we present compelling evidence highlighting the limitations of current hate speech datasets. Additionally, we conduct a range of statistical analyses to elucidate the strengths and weaknesses inherent in these datasets. This work aims to advance the development of more accurate and reliable machine learning models for hate speech detection by addressing the dataset limitations identified.
Source link
lol
Empirical Evaluation of Public HateSpeech Datasets
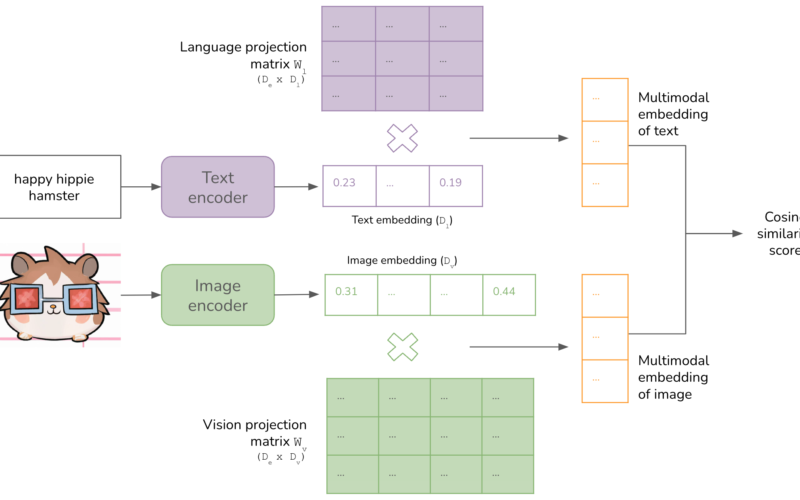